Introduction
The fundamental concept of edge computing together with its importance in contemporary tech environments needs deeper understanding by the general audience. This blog will examine edge computing in detail which delves into its fundamental components including benefits and applications and additional information.
What is Edge Computing?
A decentralized computing structure proves highly beneficial for real-time situations including smart cities platforms and IoT devices together with autonomous vehicles.
Components of Edge Computing
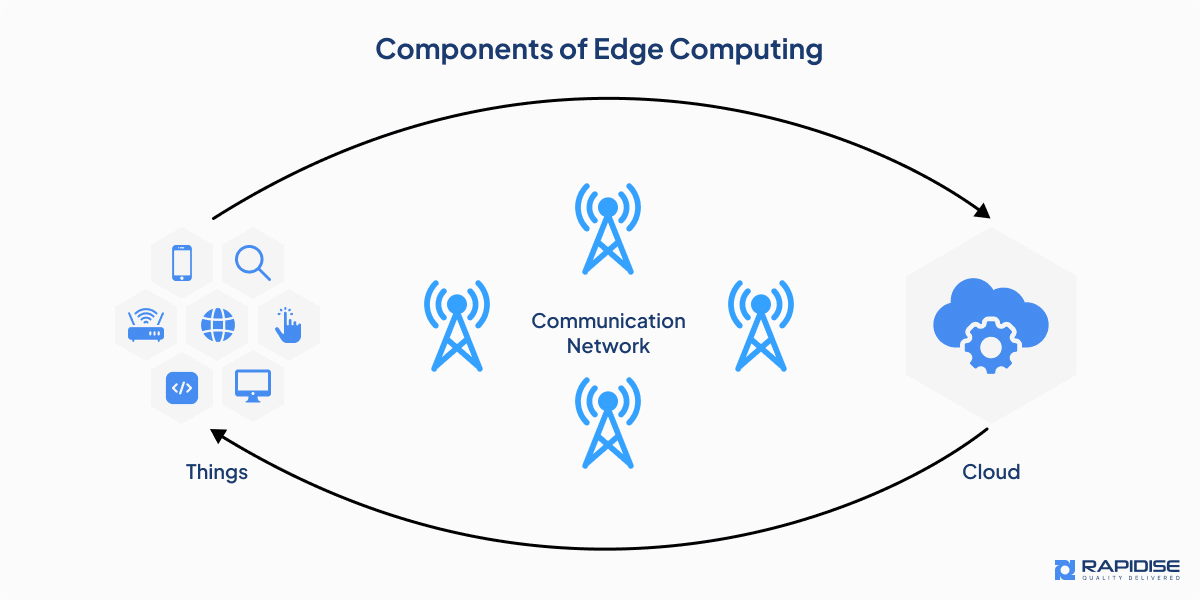
The successful operation of edge computing depends on multiple components.
Here are the primary components:
IoT
The vast amount of data generated by these devices requires instant or nearly instant processing. Process localization enabled by edge computing reduces data response time and eliminates transmission delay to environmental changes.
In a smart home configuration IoT sensors positioned on doors and windows analyze security information on-site which produces instant alarm alerts independently from cloud requirements.
Communication Networks
5G networks represent crucial infrastructure for real-time applications including autonomous driving because their millisecond response times determine safety outcomes. Communication networks provide a mechanism to connect edge data collection points with upstream analysis facilities when deeper analysis is requested.
Cloud Computing
The automation profession combines edge device response to machine data anomalies with cloud storage of historical data and predictive maintenance algorithms to achieve enhanced results. The combined strategy unites edge computing technology with cloud infrastructure to create the best possible outcomes.
Ready to Unlock the Power of Edge Computing?
Let us help you leverage the full potential of edge computing for your business.
How Does Edge Computing Work?
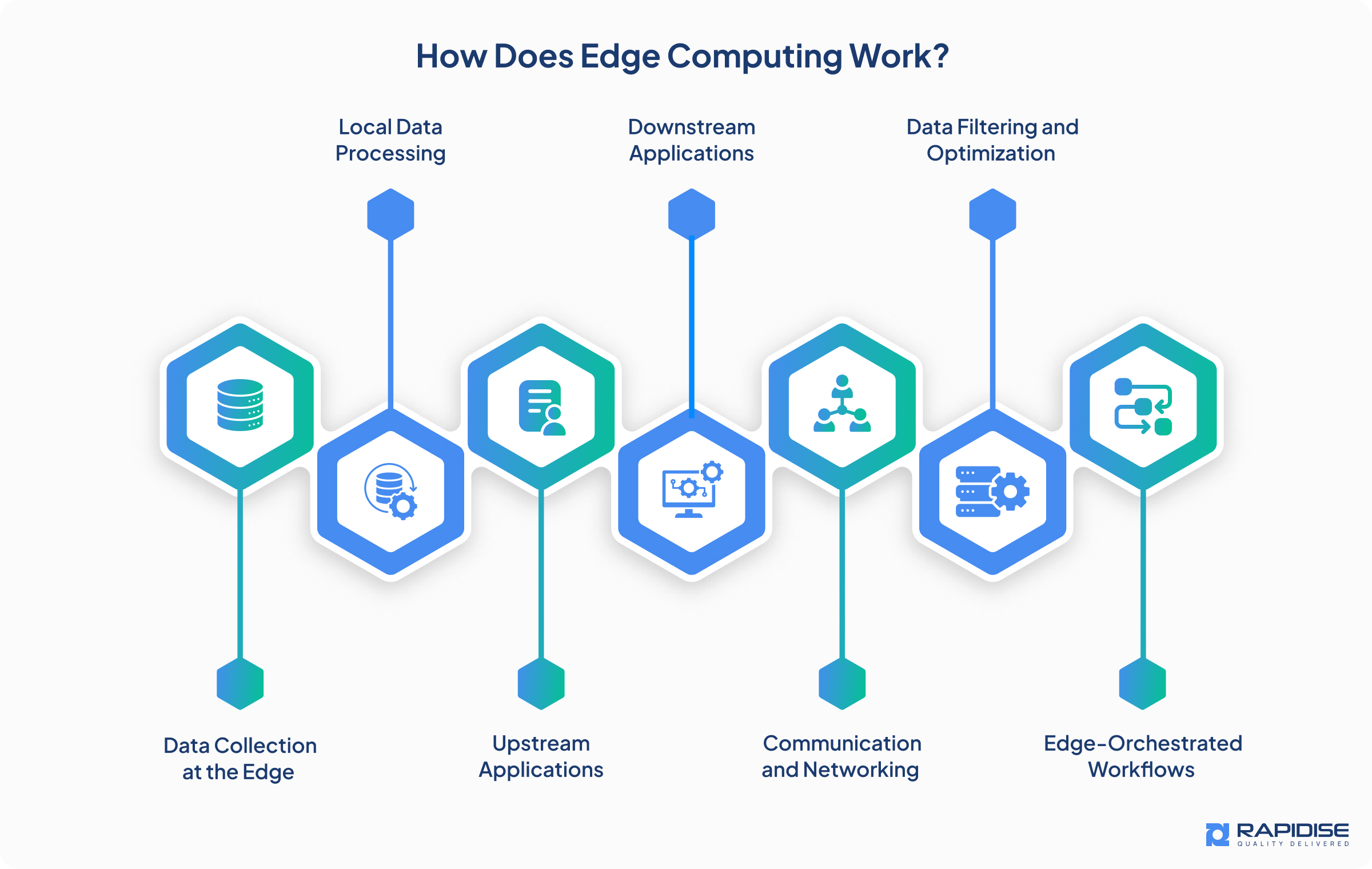
Here’s a detailed breakdown of how edge computing operates:
Data Collection at the Edge
The first elements in an edge computing architecture consist of IoT sensors along with smart cameras and industrial controllers which serve as edge devices. The devices operate in real time to gather enormous volumes of source data from their surrounding areas and mechanical systems. Industry temperature sensors work alongside urban surveillance cameras and the health monitoring wearables that provide ongoing data collection.
Local Data Processing
Edge computing security cameras evaluate suspicious activities directly on site without transferring complete video data to central storage servers. Local processing enables instant response capability when needed.
Upstream Applications
Terminated data flows in various directions toward central cloud systems and data centers for further processing purposes including analysis and archival activities preceding long-term analytics. Operational decisions happen on-site through local data analysis by factory edge devices while aggregated insights get transmitted to cloud systems for predictive maintenance and historical trend evaluation.
Downstream Applications
For example:
- Software Updates: Through cloud-based technology the system distributes updates to internet-connected devices operating at the edge of the network.
- Machine Learning Models: Machine learning models train in the cloud for deployment onto edge devices that make real-time decisions including voice command detection and manufacturing defect inspection.
Communication and Networking
The deployment of edge computing depends heavily on correct network infrastructure which implements communication protocols including Ethernet, 5G and Wi-Fi. These networks serve as the foundation for swift dependable data transfer which supports both edge-to-cloud and cloud-to-edge data movements.
Data Filtering and Optimization
Edge computing functions to decrease the volume of raw data transmission to cloud infrastructure through its capability to remove data that holds no value. For instance:
- Smart cities monitor traffic alerts for congestion by reporting deviations rather than uploading continuous structural movements to central operating systems.
- The built-in processors of a wearable health monitor review vital sign measurements onsite while warning providers about irregular readings only.
Edge-Orchestrated Workflows
Arm-based operations function as parts of comprehensive orchestrated solutions which bond different devices with cloud frameworks. Edge computing operates in autonomous vehicles by dealing with real-time sensor information for driving choices which are later combined with cloud-based driving information to enhance AI algorithms for further AI Development.
The combination of these processes enables edge computing to achieve efficient data processing that delivers both local control and centralized analytic abilities. The decentralized management system remains essential for applications that need fast reactions and execute in connectivity-limited environments.
Edge Computing vs. Cloud Computing vs. FOG Computing
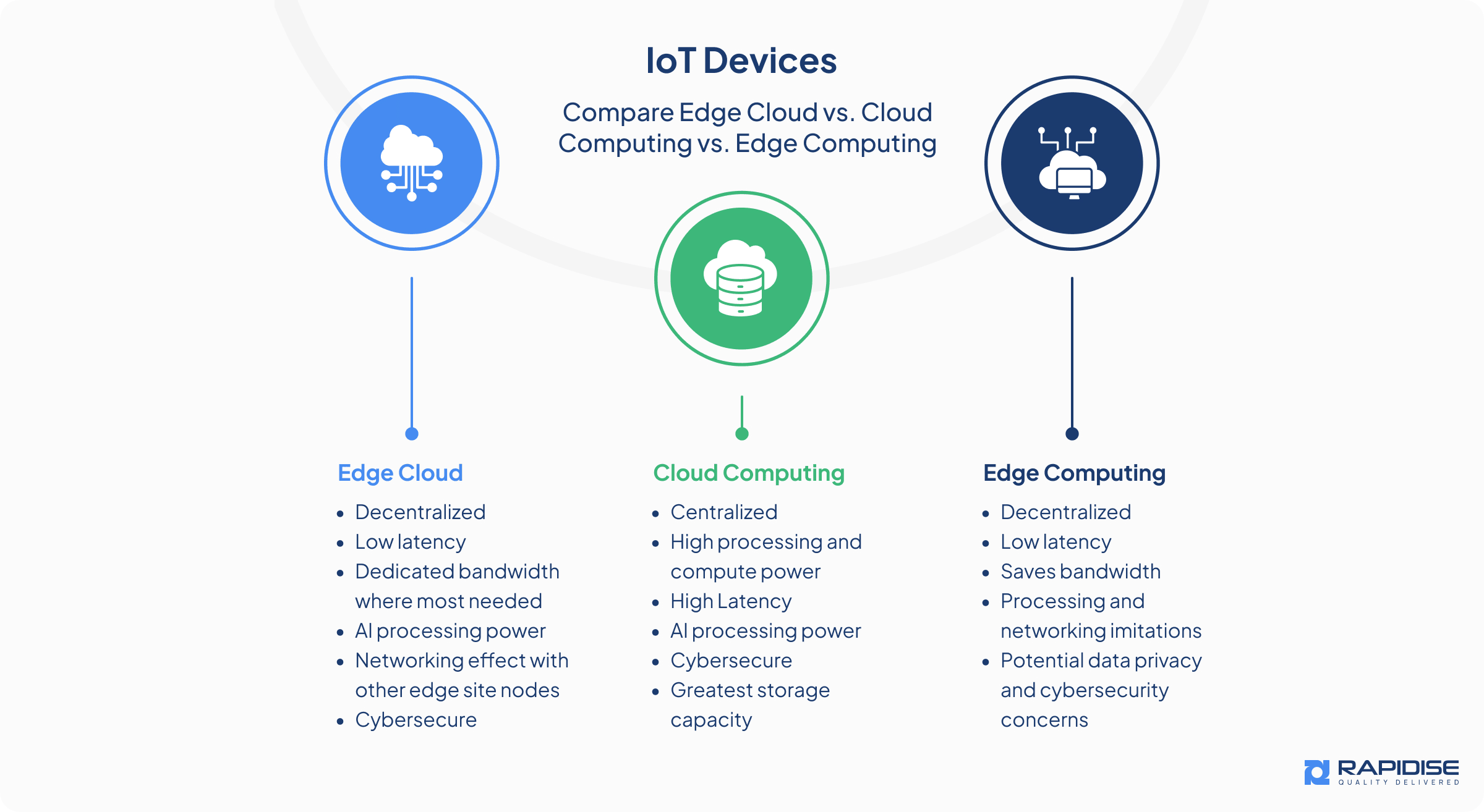
Here’s a detailed comparison of these approaches:
Edge Computing
Data processing through edge computing executes requests at proximity points to source locations which may involve IoT devices as well as edge servers. By processing data in real time edge computing decreases dependence on cloud infrastructure located in central locations.
Key Features:
- Localized Data Processing: Devices host data processing and their nearby edge servers to directly calculate information which reduces response time.
- Real-Time Responses: These applications benefit from this method because they need on-the-spot engagement in systems like self-driving vehicles combined with industrial robotics and healthcare equipment systems.
- Reduced Bandwidth Usage: Processing data locally means critical information gets streamed to the cloud exclusively thereby reducing network congestion.
- Scalability Challenges: A multitude of challenges arise in the management of numerous distributed edge hardware components.
Cloud Computing
Cloud services use remote data facility operations to centralize application processing alongside data storage functions. These services operate through the internet by offering scalable resource access.
Key Features:
- Centralized Infrastructure: Cloud servers maintain their operations from large physical data centers to deliver big infrastructure capabilities as well as abundant data storage features.
- High Scalability: Cloud frameworks adjust to changing workloads at the same time they deliver cost-efficient pay-as-you-go pricing plans to users.
- Higher Latency: The journey from source to remote data centers for processing creates delays in data delivery.
- Ideal for Long-Term Storage and Analytics: The technology satisfies diverse needs which include intelligence training alongside large-scale data evaluation together with worldwide system availability.
Fog Computing
Computing through fog functions as an intermediate layer between edge equipment and cloud infrastructure by assigning tasks to nearby network nodes instead of distant cloud facilities.
Key Features:
- Distributed Computing Layer: Fog nodes include routers along with gateways and local servers which function between edge processing and central cloud infrastructure.
- Improved Efficiency: Fog computing enables faster responses than cloud computing features while enabling edge devices to transfer specific jobs toward the cloud.
- Scalability with Coordination: The management of extensive IoT environments through fog computing functions through edge-cloud coordination systems.
- Flexibility: Edge computing systems compromise instantaneous data execution with cloud-based data storage and analytical functions.
Key Differences Between Edge Computing, Cloud Computing and Fog Computing
Aspect | Edge Computing | Cloud Computing | Fog Computing |
---|---|---|---|
Processing Location | Near the data source (on devices or edge servers). | Centralized remote data centers. | Between edge and cloud, at fog nodes. |
Latency | Minimal latency; real-time responses. | Higher latency due to long-distance data transmission. | Lower latency than cloud but higher than edge. |
Scalability | Limited by edge device capabilities. | Highly scalable with cloud resources. | Scalable with distributed nodes but requires complex coordination. |
Bandwidth Usage | Minimal; processes data locally. | High; requires transferring large data volumes to the cloud. | Moderate; balances local and centralized processing. |
Use Cases | Real-time applications like autonomous vehicles and industrial automation. | Data-intensive tasks like AI model training and global analytics. | Large-scale IoT systems like smart cities or grids. |
Importance of Edge Computing
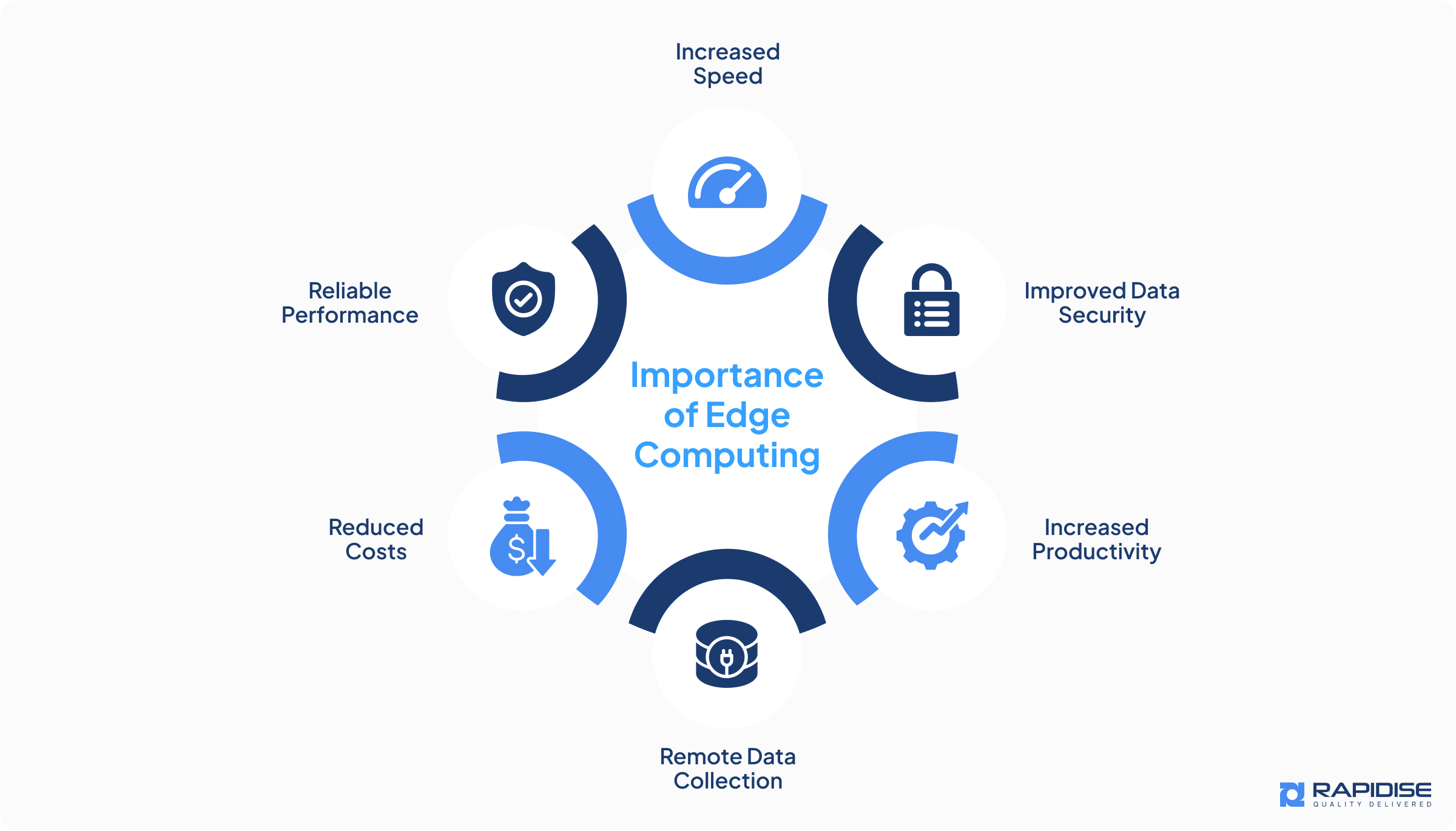
Below is a detailed exploration of why edge computing is essential and how it impacts modern technology:
Increased Speed
Commission-based computing reduces refresh times to a minimum, one of its primary benefits. The transport of data to central servers for processing constitutes a requirement of traditional cloud computing models that results in latency that affects especially operations that require real-time processing or mission-critical applications. Through local processing of data at edge computing systems organizations remove network bottlenecks while delivering instant results.
Example Use Case:
Autonomous vehicle safety depends critically on mistake-free split-second decision-making. Edge computing technology provides real-time data processing capabilities which allows vehicles to perform instant surroundings-based analysis.
Improved Data Security
Through edge computing operations maintain enhanced data security because sensitive information needs fewer transfers across extensive distances. The geographical processing location safeguards data from attackers during transit because data remains within local networks. A localized processing mechanism enables organizations to satisfy data privacy mandates including GDPR by maintaining data within precise geographical limitations.
Example Use Case:
Software programs located at the edge of healthcare systems utilize sensitive patient information from IoT-connected wearables and monitors which minimizes data exposure and allows full adherence to privacy standards.
Increased Productivity
Example Use Case:
Manufacturing relies on edge computing through real-time assessment of machine data to provide predictive maintenance capabilities. The early detection of potential problems through local data analysis helps companies keep operations running and improves overall productivity standards.
Remote Data Collection
Constraints from unstable or unreliable internet connection become a strength when edge computing technology is deployed. End devices maintain functionality in isolated regions because they conduct their own data processing and storage operations without network dependence.
Example Use Case:
Remote agricultural IoT systems leverage edge computing technology to keep tabs on soil conditions as well as weather and crop wellness through real-time monitoring so local farmers can make informed choices while facing intermittent internet access.
Reduced Costs
The elimination of dependence on cloud systems produces cost reduction potential for organizations through reduced expenses related to data movement and processing and data storage. The local data filtering capabilities of edge computation enable businesses to redirect critical data for processing at the source location and diminish cloud bandwidth requirements and storage expenses.
Example Use Case:
Retail businesses that use edge devices in their stores can process purchase transactions together with inventory modifications within local boundaries, so they lower both cloud expenses and continue regular retail operations.
Reliable Performance
The system design of edge computing maintains dependable system functioning when operating in networks with unreliable Internet connectivity. Local data processing capabilities allow systems to execute operations independently of continuous connection to centralized data facilities.
Example Use Case:
Local data processing in energy management systems enables smart grids to distribute energy better while continuing stable operations independently during internet downtime.
Edge Computing Combined with Other Technologies
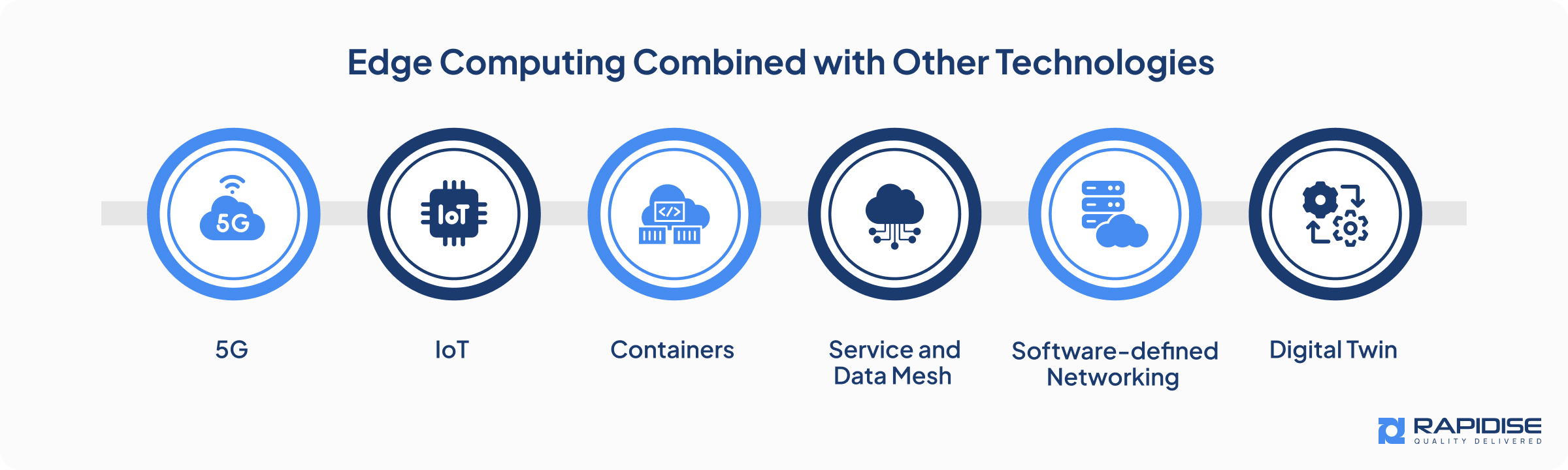
All of edge computing’s real power emerges from its natural combination with advanced technological systems. The combination of edge computing with other advanced technologies produces improved operational efficiency alongside scalable capabilities and enhanced industry innovation. Let’s explore how edge computing works alongside various technologies and their combined impact:
5G
A breakthrough development occurs through the combination of 5G with edge computing which yields both high-speed data transfer and minimal response delays.
Benefits of Combining Edge Computing with 5G:
- Low Latency: The 5G network establishes momentary information exchanges between edge servers and devices.
- Higher Bandwidth: The platform enables efficient operation for processing extremely large IoT data volumes.
- Enhanced Applications: The combination of 5G technology enables vital applications including autonomous vehicles and augmented reality (AR), virtual reality (VR) and smart cities.
Example Use Case:
Gaming and remote training applications supported by 5G and edge computing networks deliver smooth high-speed performance for entirely immersive experiences that bypass lag.
IoT
The rate of data generation from IoT devices requires fast processing methods which enable real-time analytical capabilities. Through edge computing IoT data is processed efficiently at its operational source thus minimizing dependence on distant cloud infrastructure.
Benefits of Combining Edge Computing with IoT:
- Improved Efficiency: Analytics performed directly on data source locations speeds up processing times.
- Enhanced Scalability: The solution enables increased scalability while keeping IoT devices from straining cloud capacities.
- Real-time Decision Making: The system delivers rapid instantaneous responses needed for crucial healthcare applications and industrial automation operations.
Example Use Case:
Edge computing together with IoT sensors enables smart homes to process data at its location for live adjustments of security settings, temperature control and indoor lighting.
Containers
The lightweight yet portable container system enables application deployment across devices including edge computing platforms to become simpler. Edge computing when combined with containers allows for manageable application deployment across distributed platforms and flexible resource scaling capabilities.
Benefits of Combining Edge Computing with Containers:
- Portability: A consistent application execution environment becomes possible across all edge devices even when running on different hardware configurations.
- Scalability: New application deployment becomes easier and faster because containers allow seamless distribution to edge nodes throughout their network.
- Resource Optimization: Containers as a technology utilize edge device resources more effectively through application isolation within defined containers.
Example Use Case:
AI-driven recommendation engines deployed through containers at retail store edge devices ensure local customer-specific experiences while avoiding server cloud saturation.
Service and Data Mesh
Significant improvements in edge computing data management flow from adopting service mesh alongside data mesh architectural elements. The distributed nature of edge computing finds exact alignment within data mesh framework because this architecture decentralizes data management, yet service mesh provides microservices communication support.
Benefits of Combining Edge Computing with Service and Data Mesh:
- Improved Communication: A service mesh creates secure traffic pathways between applications operating on devices in edge environments.
- Decentralized Data Management: By implementing data mesh organizations can locally control their data which decreases their dependency on single centralized systems.
- Enhanced Flexibility: Edge networks become simpler to operate when multiple computing nodes connect inside one network.
Example Use Case:
The connectivity of service mesh allows simple data exchange between urban devices which monitor traffic together with energy and public security systems in extensive smart cities.
Software-defined Networking
SDN splits network functionalities into control plane functions and data plane functions which allows greater flexibility in resource allocation via automated systems. SDN optimization of data transmission happens when it connects to edge computing networks and core infrastructure.
Benefits of Combining Edge Computing with SDN:
- Flexible Network Management: This technology responds immediately to shifting requirements encountered in edge computing ecosystems.
- Improved Traffic Optimization: The system uses efficient data routing to lower latency in the network.
- Simplified Network Configuration: Centralized network management becomes possible through this implementation while providing distributed networks with scalability benefits.
Example Use Case:
Telco companies leverage SDN edge computing technology to control traffic flow which maintains continuous service quality through busy times for their subscribers.
Digital Twin
A digital twin represents an exact virtual copy of actual physical systems alongside their connected processes. The convergence of digital twins with edge computing grants users access to real-time data evaluation as well as condition simulation and system optimization capabilities.
Benefits of Combining Edge Computing with Digital Twin:
- Real-time Insights: Real-world data undergoes processing at the edge devices which allows instant digital twin updates.
- Predictive Analytics: The system uses processed edge data to recognize potential breakdowns then proposes remedial methods.
- Cost Savings: System performance maintains security because real-time updates and monitoring happen outside cloud environments.
Example Use Case:
Edge computing enables manufacturing digital twins to analyze machinery data while simultaneously predicting maintenance requirements and optimizing production flow in real time.
Edge Computing Benefits
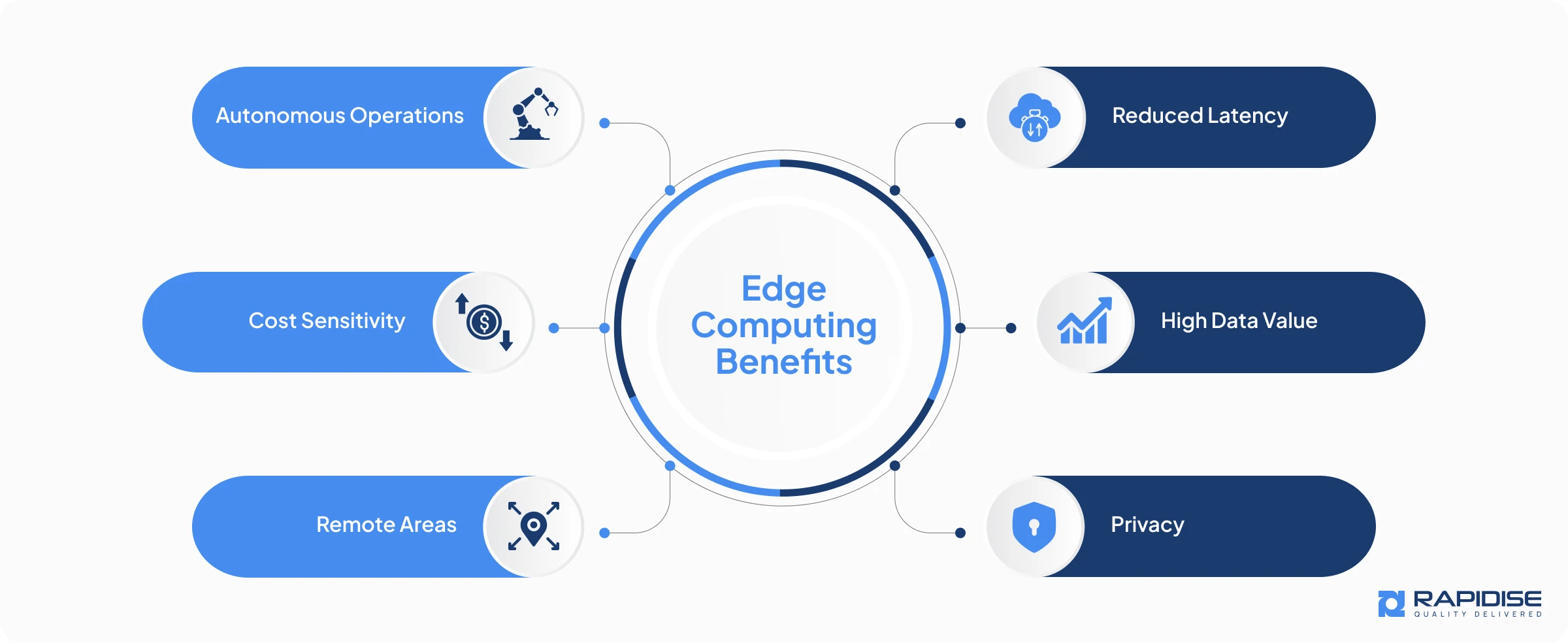
Here is an expanded view of the key benefits:
Reduced Latency
Auto vehicles benefit from reduced latency because it lets them process data rapidly which improves both operational performance and driver safety. Through localized processing operations, you can achieve better response times and provide consistent user experiences for multiple industries.
High Data Value
Hardware located at patient points can utilize edge computing to examine data obtained from wearable devices which then creates immediate alerts for healthcare professionals. The implementation of localized processing enables companies to take action on data precisely at the moment when it becomes most effective.
Privacy
Edge computing establishes essential protection measures for finance sector data security through local data handling that follows privacy regulations effectively. Through localized approaches the system develops user trust and simultaneously fortifies cybersecurity systems as a whole.
Remote Areas
In rural agricultural areas edge computing empowers IoT devices through localized data processing for soil condition and weather pattern observation which helps farmers make better decisions. Through its decentralized processing power edge computing delivers technological advantages to areas bypassed by infrastructure while fostering both inclusive development and social advancement.
Cost Sensitivity
Retail applications that perform on-site customer analysis through edge computing methods can achieve operational expense reduction by cutting down cloud data transfers. Organizations that localize their data storage and processing activities achieve budget optimization without compromising on performance results.
Autonomous Operations
Important facilities such as oil rigs and offshore wind farms and remote mining operations achieve real-time monitoring capabilities together with control systems by employing edge computing since they lack consistent internet connectivity. The capability to operate independently heightens reliability while allowing uninterrupted operations that decrease equipment downtime during difficult environments of limited connectivity.
Explore Innovative Edge Computing Solutions
Looking for a reliable partner to transform your business with seamless edge computing integration?
Edge Computing Challenges and Opportunities
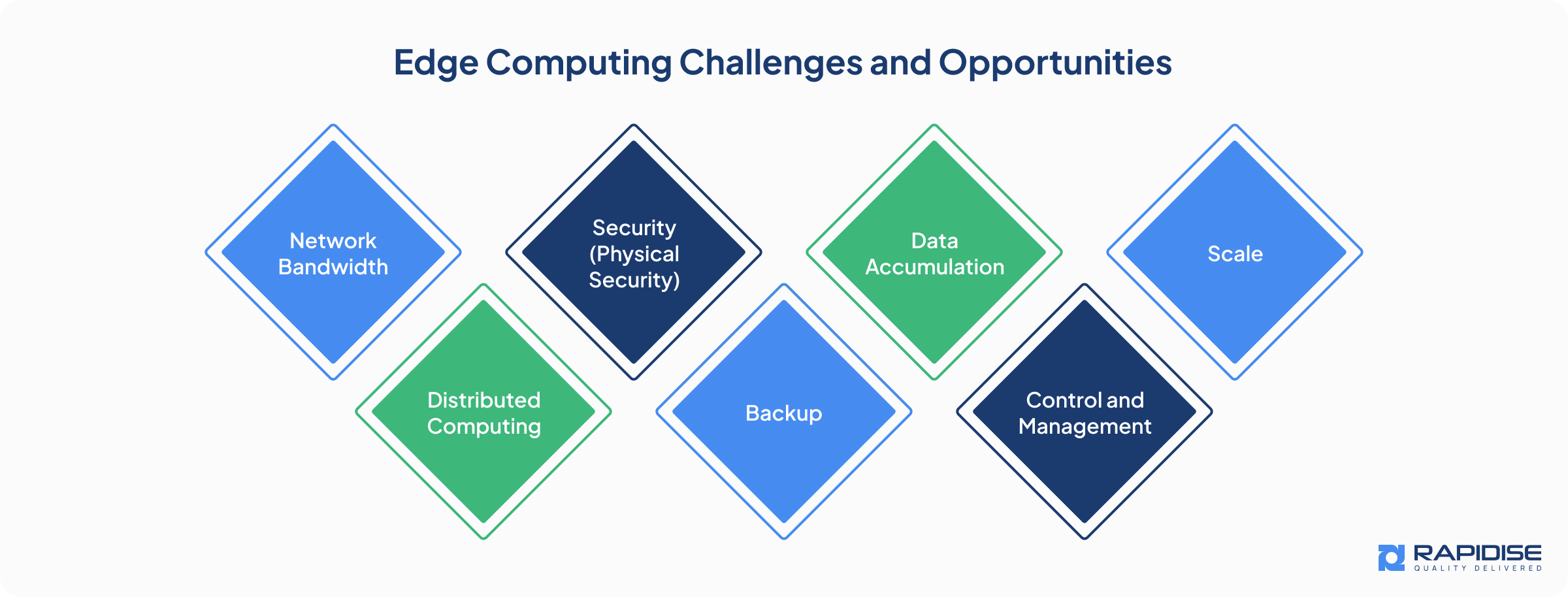
The deployment of edge computing brings many benefits to business organizations, yet companies need to solve various implementation difficulties. The obstacles facing edge computing systems open doors for businesses to develop innovative solutions and expand operations.
Network Bandwidth
The constraints in edge processing demand new networking technologies like 5G together with low-power wide-area networks (LPWAN) for faster data transfer and minimized latency. Investing in edge-native networking systems creates new possibilities in data-driven applications while solving bandwidth constraints faced by businesses.
Distributed Computing
The difficulty presents the chance for developing advanced edge orchestration tools alongside AI-driven automation frameworks. Organizations apply machine learning and software-defined networking technology to distribute computing workloads which achieves higher efficiency together with enhanced reliability.
Security (Physical Security)
The solution includes creating advanced encryption techniques as well as biometric verification systems and tamper-resistant hardware systems. Fortified edge security protocols provide dual advantages of protecting sensitive information and building both client trust and industry-sector compliance especially in sectors including healthcare and finance.
Backup
A challenge emerges for creating dispersed backup systems based on blockchain protocols and distributed database approaches alongside redundant storage frameworks. Artificial intelligence enabled data replication strategies improve business continuity during hard times by building resilient data storage systems.
Data Accumulation
The challenge enables possibilities for AI-driven data filtering methods combined with automated analytical tools and storage tiering solutions which enhance data process management. By finding ways to address their data accumulation businesses can make better decisions while controlling data storage expenses.
Control and Management
Elliptical managers require centralized edge control solutions which establish an opportunity for AI-based monitoring platforms. The integration of automated deployment with predictive maintenance capabilities and self-healing systems will transform edge computing management through increased scalability and decreased costs.
Scale
The difficulty of scaling edge computing promotes the development of frameworks which combine modularity with interoperability. Standard edge computing solutions that include open-source platforms with cloud-native architectures help businesses implement easy expansion of their edge computing operations across locations and industries.
How Can Rapidise Help You with Your Edge Computing Requirements?
From IoT device deployment to real-time analytics operationalization and AI automation establishment, our team provides sustained support for reaching your organizational targets. Partnering with Rapidise grants you immediate access to current technological solutions and expert implementation best practices with staff who specialize in edge computing complexity management.