Introduction
The process of data handling through edge computing takes place at the original source as an alternative to remote cloud server systems. AI collaborations enable new breakthroughs which minimize delays while improving protocol protection systems and data stream management and provide system reliability. This blog examines the major advantages of edge computing for AI programs alongside its role in defining future operational capabilities of intelligent systems.
1. Latency Reduction and Real-Time Processing
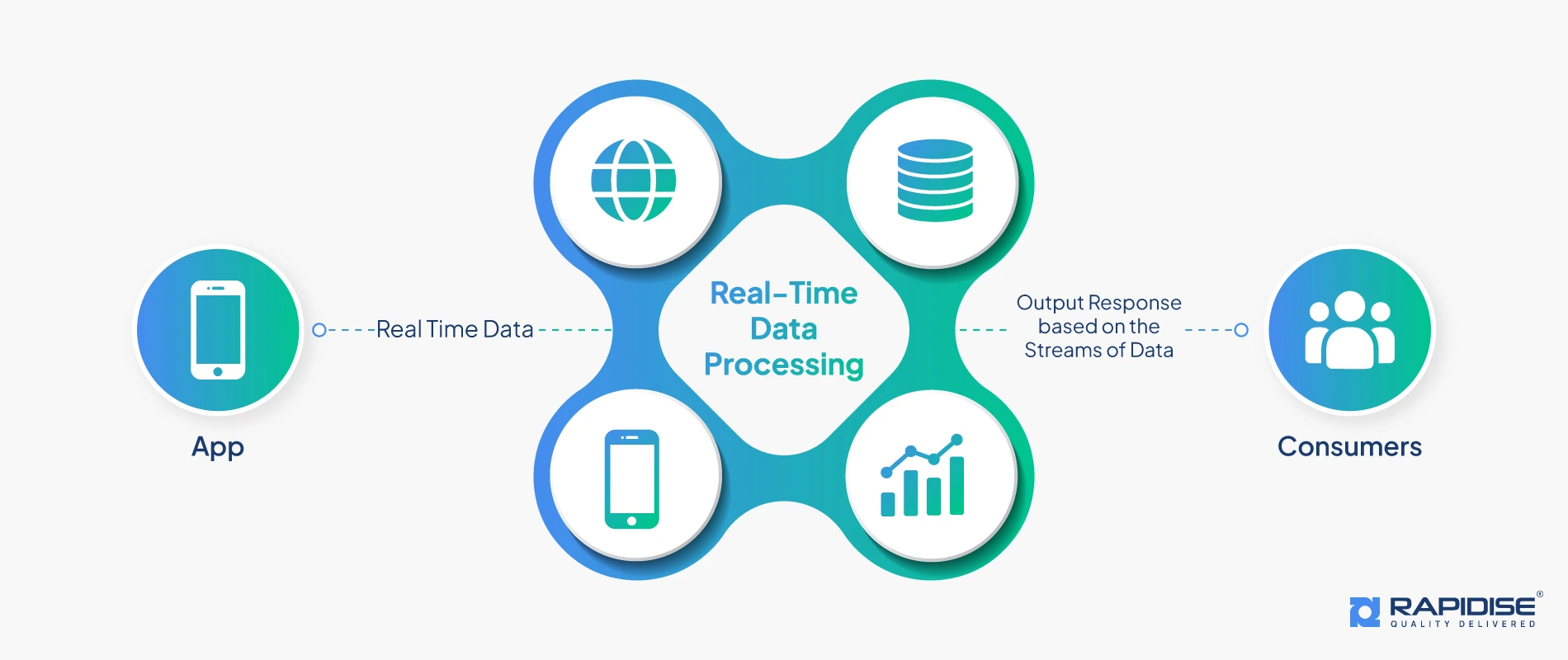
The main limitation of implementing AI through cloud services is performance slowness. The real-time needs of AI applications suffer when data must be transmitted to cloud servers for analysis because this procedure creates performance-degrading delays.
The Problem of Latency in Cloud-Based AI
The execution time of cloud-based AI becomes an issue when real-time processing needs exist among applications like autonomous vehicles or industrial automation systems as well as healthcare services. Response times suffer from the combined effects of distant physical data connections and limited network bandwidth as well as server-to-cloud distances which render cloud AI inappropriate for time-sensitive decision tasks.
How Edge Computing Minimizes Latency
Through edge computing AI processing receives improved efficiency because AI applications operate via edge devices consisting of IoT sensors along with gateways and embedded systems near the data sources. The data analysis process at localized locations becomes faster in this scenario while simultaneously eliminating the delay which comes from cloud-based operations. Edge AI processes data proximity to its source location to yield swift decisions alongside quick response times that critical time-sensitive operations demand.
Real-World Examples:
Autonomous Vehicles:
Self-driving vehicles need to analyze vast quantities of sensor information immediately to both sense traffic elements and navigate through quick thinking decisions. Deploying AI initiatives through cloud services involves delays that create safety risks for accidents to occur. Edge computing allows AI-powered systems to operate on-board vehicles because it delivers quick low-latency choices that create safer autonomous driving operations.
Industrial Automation:
The predictive maintenance systems in manufacturing environments utilize AI to monitor equipment which allows identification of future faults before they start. Real-time anomaly detection through traditional cloud AI systems cannot be achieved because of delays caused by latency. An edge AI system analyzes sensor data straight away to detect performance deviations thus it provides quick responses which decreases manufacturing downtime expenses.
Remote Surgery:
Remote surgery requires minimal latency because surgeons use remote robots to operate on patients through such systems. The processing time of transmitted data needs to be immediate to avoid medical complications during various surgical procedures. Through its edge computing approach image processing and force feedback methods and motion control interface in time for exact and punctual surgical actions.
Boost Your AI Performance through Edge Computing Solutions
The business-specific Edge AI solutions by Rapdise enable secure operations and minimize expenses while eliminating delays.
2. Enhanced Privacy and Security
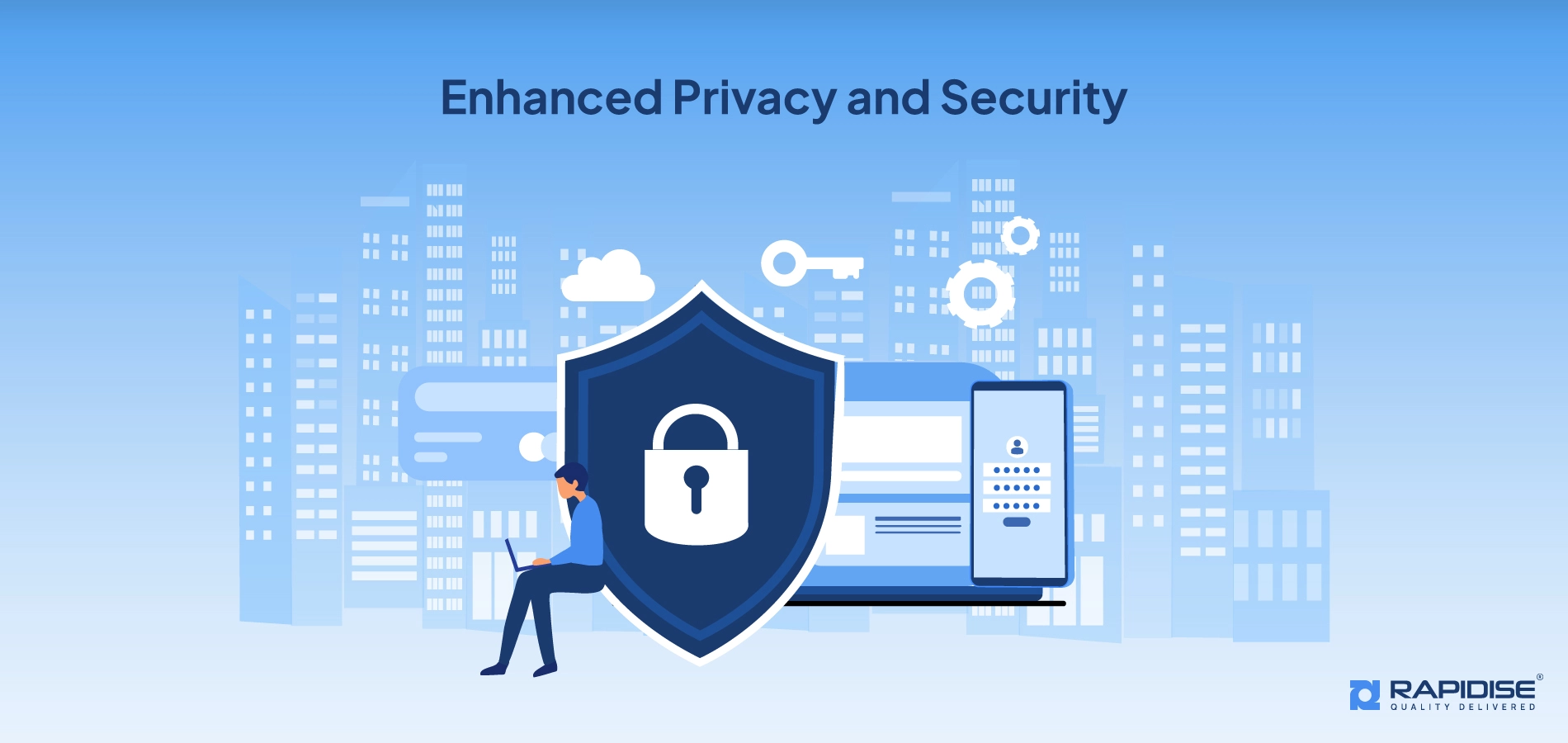
Security becomes a primary issue in cloud data transfers of sensitive information. Data protection becoming a serious issue for AI systems connected to cloud infrastructure leads to both security breaches and legal failures regarding noncompliance.
Privacy Concerns with Cloud-Based AI
Organizations face difficulties in meeting GDPR and CCPA data protection requirements when handling sensitive client data which must be transmitted and stored on single cloud-based servers. A solution must exist to reduce data exposure level but still meet regulatory compliance standards.
How Edge Computing Enhances Data Privacy
The process of data anonymization and encryption at local levels stands as another protection measure before any required cloud transmission occurs. Organizations that conduct data processing at its source maintain compliance with data privacy regulations because they avoid violating strict privacy laws and regulatory requirements.
Security Benefits of Reduced Attack Surface Area
The risk reduction of edge computing becomes possible through its design which spreads service across several edge devices rather than having one centralized point of storage. Decentralized edge networks create effective obstacles for hackers who try to execute large attacks because one hacked edge device does not cause the same damage as a compromised cloud center.
Through local processing at the edge computing nodes businesses eliminate their excessive dependence on permanent cloud connection which safeguards operations against service outages and cyberattacks on cloud systems. Operational continuity and security enhancement become possible for businesses through executing AI workloads on local resources because of cloud service disruptions.
Compliance with Data Privacy Regulations
Edge computing lets businesses fulfill compliance standards while keeping control of sensitive information through its data processing methods that operate inside local storage environments.
Real-World Example: Processing Biometric Data Locally
Biometric data processing through edge computing takes place directly on secure edge devices such as smartphones also smart access control systems where personal identifiers always stay confined within the device hardware. Security measures are strengthened by this method while users gain better privacy outcomes together with improved trust.
3. Bandwidth Optimization and Cost Savings
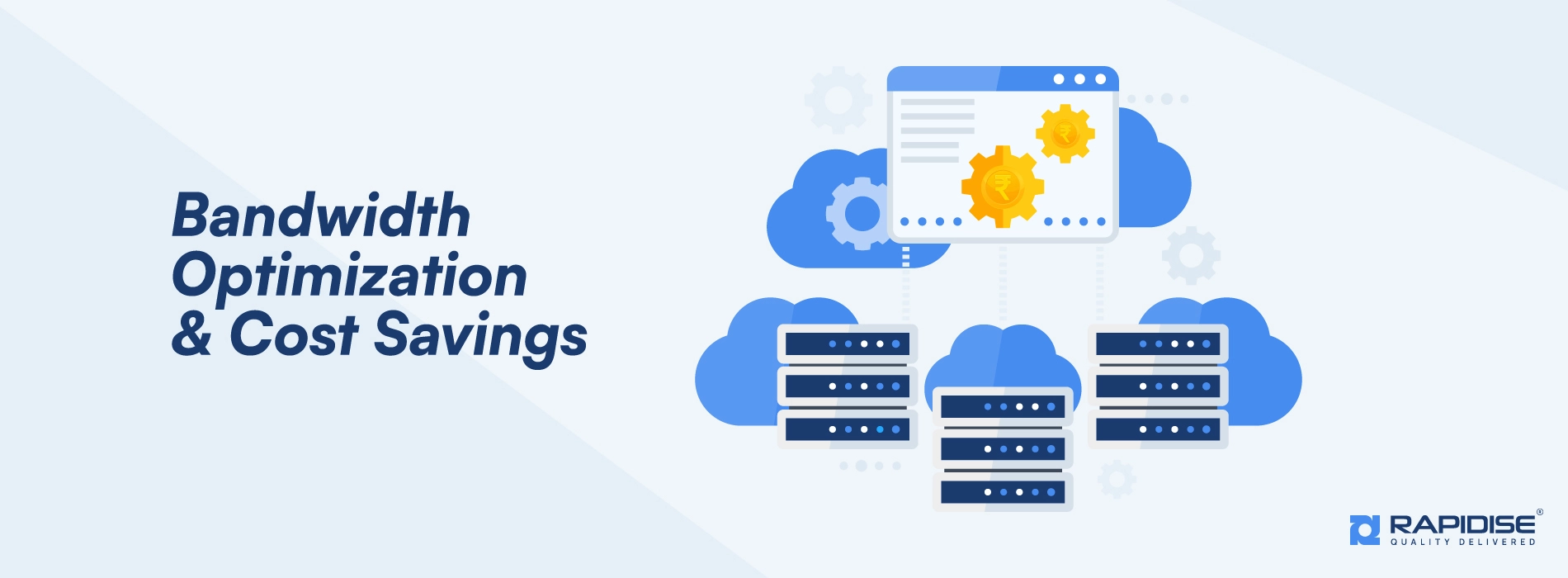
Cloud-based AI systems need to transport sensitive information across the internet for computing purposes which creates hazards from cyber intrusions as well as unauthorized exposure and difficulties in satisfying regulatory standards. The secure nature of Edge computing emerges because it processes information near its actual location which reduces attack possibilities and maintains strict privacy rule compliance.
Privacy Concerns with Cloud-Based AI
Large cloud-based data centers become preferred targets of cybercriminals due to their massive storage of valuable information from users. The exposure of personal and confidential data during network publication leads to possible interception of such information.
Organizations face significant challenges regarding both the location of their data and their compliance with privacy regulations that include GDPR and CCPA because of their strict requirements.
Organizations encounter substantial challenges in complying with regulatory requirements while using AI in the cloud because offshore cloud servers create legal difficulties with data storage and processing. Organizations which manage vulnerable data must acquire a system that both protects privacy rules and reduces potential data exposure.
How Edge Computing Enhances Data Privacy
Storing personal data as well as sensitive information inside protected network zones keeps them safe from potential exposure risks. The local processing of data enables businesses to apply security measures from data anonymization and encryption before they send vital insights from the processing station to the cloud servers.
Through this method organizations achieve better protection of their user data because they maintain direct administrative control thus minimizing unauthorized access risks. Organizations that need real-time AI analytics support in healthcare along with finance and defense fields can protect sensitive information during data usage for AI-driven insights.
Security Benefits of Reduced Attack Surface Area
Through edge computing data processing spreads across various edge devices so attackers find it more difficult to perform major system breaches. The consequences of edge device breaches remain confined to one device despite hacker intrusion because the system is not affected as a whole.
The implementation of edge AI eliminates the need for constant connection to internet which prevents man-in-the-middle attacks and cloud-downtime and network threats from affecting systems. The processing of data at its source point makes businesses less exposed to disruptions resulting from cyberattacks which target cloud systems. The decentralized processing method strengthens system resistance and creates better operational security features.
Compliance with Data Privacy Regulations
EU businesses that operate under GDPR must maintain personal data belonging to EU citizens within data protection laws that ensure adequate privacy in their operational territories. Edge computing technology enables local AI model deployment on EU-based servers or devices which allows businesses to manage data within national boundaries through local processing. Financial institutions together with healthcare providers utilize edge AI technology to follow HIPAA regulations by keeping patient records inside protected on-site systems.
Real-World Example: Processing Biometric Data Locally
Through edge computing biometric data gets processed directly on secure devices such as smartphones and access control systems and surveillance cameras and thereby personal identifiers remain contained within the local device.
Users can enjoy facial recognition through Face ID and Pixel Face Unlock because these systems rely on edge AI processing at the device level. Device users maintain full system security through data being processed directly on their devices without cloud upload functions. Companies using AI security systems in airports and corporate offices should deploy local facial recognition within their operational sites to minimize data risks during authentication procedures.
Future-Proof Your AI Applications with Edge AI
Our Edge AI solutions bring intelligence closer to your data source, ensuring ultra-low latency, better reliability, and real-time decision-making.
4. Improved Reliability and Availability
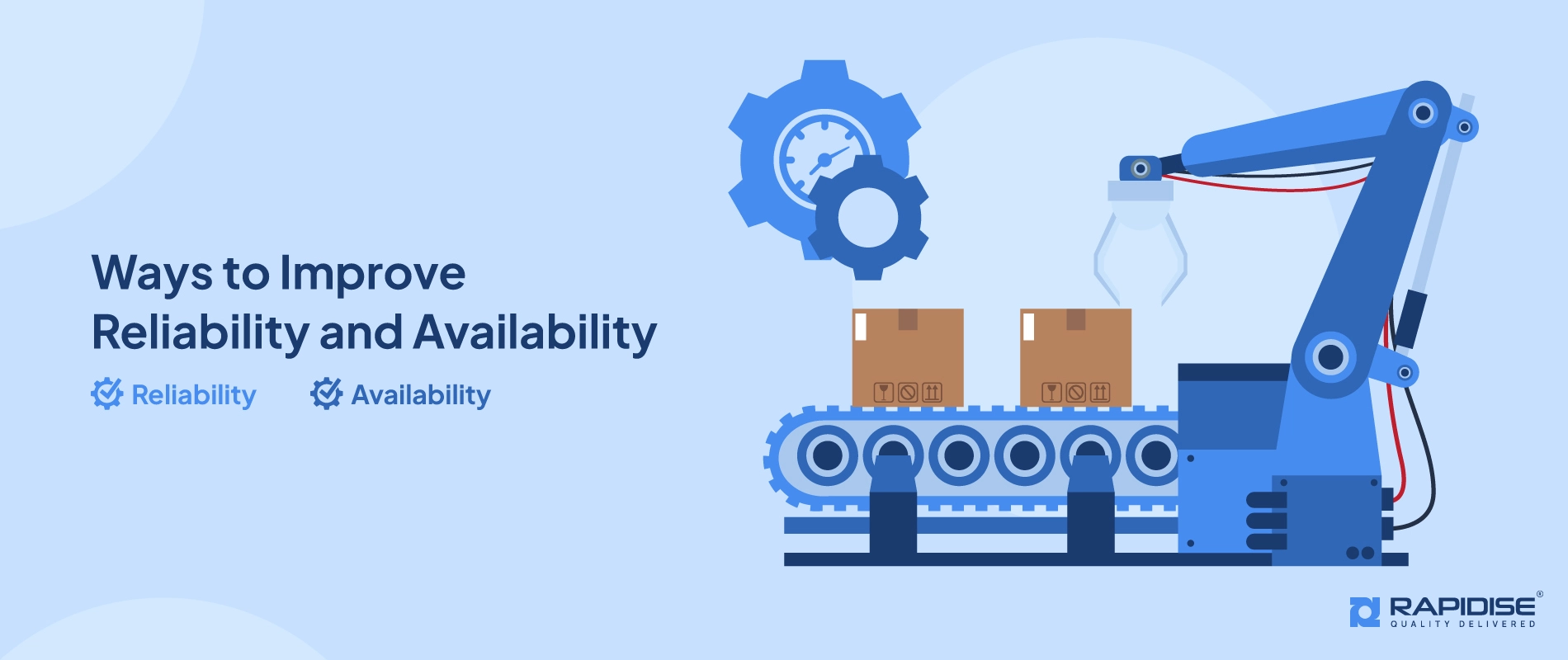
The main weakness of cloud-based AI systems results from their necessary Internet connection and their centralized data storage systems. System interruptions and lost network connectivity within cloud services deactivate AI applications which then causes interruptions during essential operations. The functionalities of edge computing fix these problems by moving AI operation away from central points and enabling basic operations when cloud disruptions occur.
Single Points of Failure in Cloud-Based AI Systems
A real-time AI-driven manufacturing system depends on cloud servers for equipment failure detection but it will experience both delayed performance and complete system stoppages when an internet connection fails. Such conditions could lead to equipment malfunctions that trigger stopped productions which subsequently causes economic damage. When cloud outages occur the AI-powered traffic management system for smart cities would become nonfunctional thus resulting in safety hazards combined with traffic congestion.
Dependence on cloud-based AI creates one vulnerable point in business operations which disrupts vital application systems. Distributed AI processing across numerous edge devices through edge computing brings independent operation of separate components in case of one failure.
How Edge Computing Creates a More Resilient and Distributed System
Thanks to its distributed architecture an edge computing system maintains continuance of network operation when a single device faces problems. Edge-based sensors and cameras in retail stores with AI customer analytics functionality will continue processing and gathering data locally whenever the connection to the cloud becomes lost. When connection returns the system integrates with the cloud storage but continues providing uninterrupted real-time decisions.
Edge computing performs workload distribution through load balancing since available edge devices automatically receive AI processing responsibilities dynamically. Throughout high-demand times AI applications continue their responsiveness since device distribution across available computing units prevents system throughput interruptions.
Edge Devices Operating with Intermittent or No Cloud Connectivity
Remote monitoring systems that use artificial intelligence for oil rigs and offshore wind farms encounter restricted internet connectivity while performing their tasks. Such systems benefit from edge computing because they maintain continuous data processing of sensors at their local level for finding irregularities and forecasting equipment breakdowns independently of network connections.
Detection of major issues enables the system to transmit critical alerts to the cloud as a method to optimize bandwidth utilization while enabling prompt actions. The combination of edge AI technology allows autonomous drones participating in search and rescue operations to both analyze video data in real-time and recognize objects and run navigation algorithms on a device level.
The ability for drones to operate in disaster areas without internet connection increases their effectiveness while reducing emergency response time and improvement of rescue operations. AI controls security surveillance systems which operate within high-risk areas. Vehicle bases alongside border control stations and secluded research sites need uninterrupted security observation.
Edge AI surveillance cameras evaluate live videos instantly to detect security risks and detect unusual motions at the camera’s local position. These systems maintain their ability to detect and respond to security threats independently when cloud connections fail because of their local operations capabilities.
5. Scalability and Flexibility
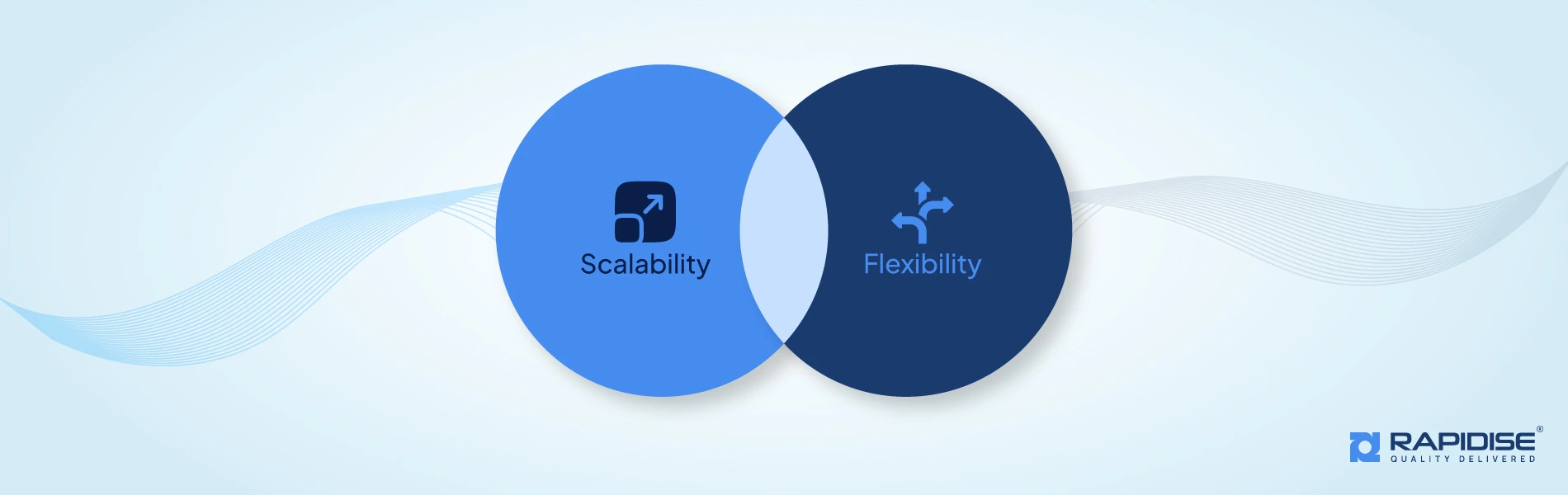
Businesses and developers need efficient adaptable and scalable solutions to deploy AI models in different computing environments because of increasing AI application complexity and scale demands. The current architecture of cloud-based AI finds itself incapable of handling large-scale deployment requirements mainly during real-time data processing together with distributed AI workloads. The advantages of edge computing include its ability to expand with ease and provide operational flexibility and support all different hardware and software frameworks.
Easier Scaling of AI Deployments with Edge Computing
Edge devices have the ability to distribute AI processing operations between them thus relieving centralized cloud facilities of performance responsibilities. Organizations can straightforwardly increase their AI capabilities because they avoid substantial investments in cloud storage together with processing power.
Local AI model deployment to edge devices together with IoT sensors and processing units grants businesses the power to make their AI applications scale properly while staying beneath network capacity thresholds. AI-powered customer analytics operated by retail chains at multiple stores functions best by running local computations at store locations instead of forwarding all data to centralized cloud storage. The system supports time-sensitive decision-making processes at all locations while decreasing expenses for cloud network equipment.
Deploying AI Models Across a Large Number of Edge Devices
Self-driving cars together with autonomous drones utilize edge AI models that need deployment across thousands to millions of vehicles for updating purposes. Edge-based AI processing enables data analysis of each vehicle directly at the local system while controlling the need for cloud transmission. AI powered vehicles maintain autonomous operation through this approach because they do not face timing issues stemming from cloud-based transmissions.
Medical devices benefit from edge computing when AI-powered healthcare applications use the technology to distribute models over numerous diagnostic instruments and wearable health monitors. The ability to monitor health conditions personally and in real time reduces cloud connectivity needs because patients obtain immediate health alerts directly.
Flexibility of Edge Computing in Supporting Diverse Hardware and Software Platforms
AI-powered smart factories implement an optimization system by connecting IoT sensors with AI-powered cameras and robotic arms and edge servers located at the production area. The different hardware capacities and software specifications of these devices become feasible to run AI models through edge computing technology. Specific needs of businesses determine their AI implementation without facing limitations from standardized cloud options.
Edge computing enables AI model inference to run on different hardware systems that include ARM-based chips for mobile devices in addition to NVIDIA GPUs utilized for industrial systems as well as the specialized AI accelerator Edge TPU developed by Google. AI deployment across different business environments becomes possible because organizations avoid concerns about compatibility issues.
Example: Rapid Deployment of AI Models Across a Fleet of Connected Devices
Real-time AI data processing provides giant delivery companies with necessary insights to optimize routes and monitor driver safety and predict vehicle system failures when they operate a large fleet of delivery trucks. Edge AI functions directly from computing units inside vehicles at identical locations to cloud-based AI solutions which suffer from operational delays.
Each vehicle operates its edge AI system directly on board through the combination of GPS data and vehicle diagnostics in addition to driver performance metrics for rapid instant decision-making capabilities.
The system sends vital data to the cloud during emergencies only to minimize both operational expenses and to uphold system performance levels. New AI model updates are installed effortlessly into the entire fleet because edge AI systems perform this deployment independently from cloud connectivity requirements.
Scale AI Faster with Edge AI Optimization
Our expert team helps you implement optimized AI models, secure edge infrastructure, and scalable deployment strategies to keep your business ahead.
AI Application Use Cases
Across multiple business sectors this combination enables new types of solutions. Multiple industrial sectors have experienced revolutionary change through edge AI because it enables fast processing and reduced delay time and enhanced security and optimized bandwidth management. Several business entities and organizations use edge computing to drive AI applications through powerful implementation scenarios.
Smart Cities:
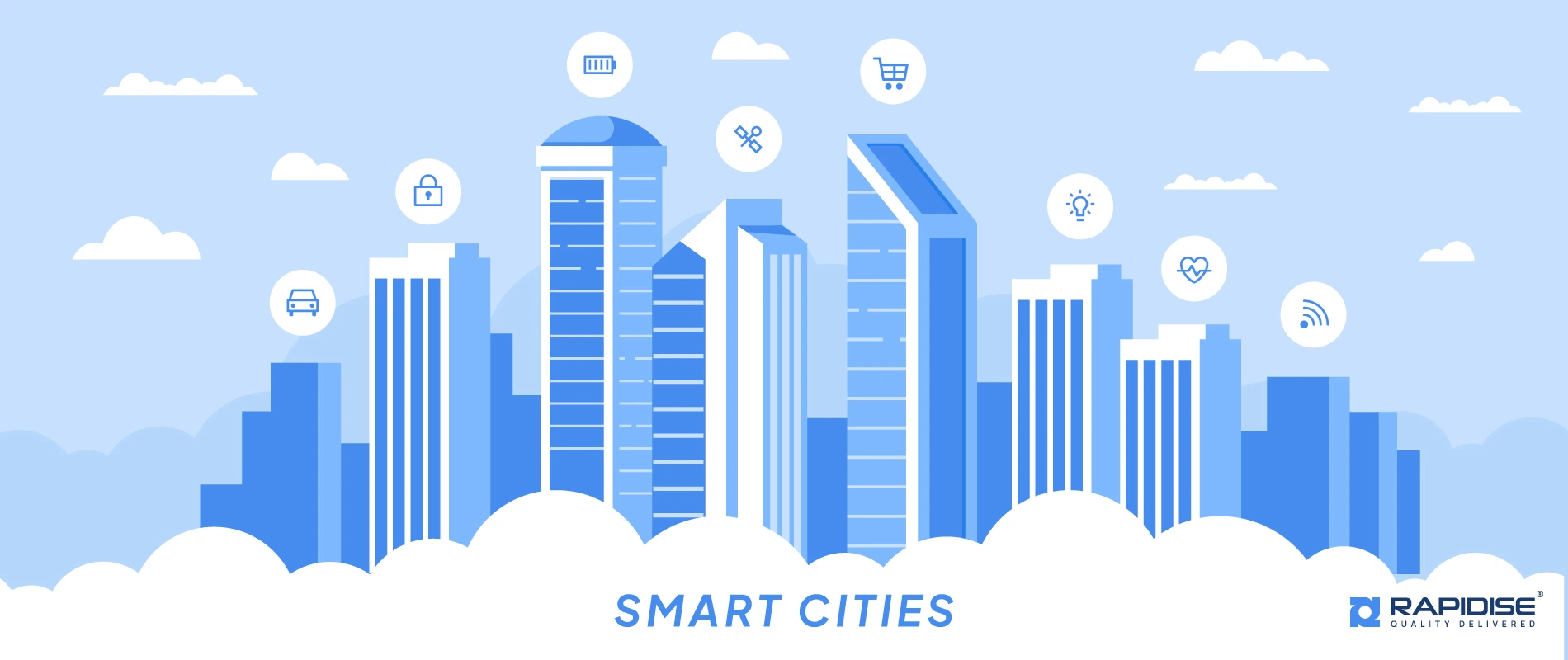
Traffic management systems based on AI use edge computing to process data collected from traffic cameras and IoT sensors as well as connected vehicles to control signals for congestion reduction. The systems collect real-time information about accidents with the addition of bottlenecks and traffic violations so authorities can intervene immediately instead of relying on cloud-based analytics.
Edge AI-enabled sensors deployed in environmental surveillance systems monitor performances of air quality along with temperature measurements and noise pollution ratings and water contamination levels. Mutual air quality monitoring devices manage their data processing onsite which enhances municipal ability to make fast decisions about pollution incidents independently from cloud networks.
Edge AI demonstrates significant importance in public safety by improving security operations. AI-based security cameras that embed microprocessors at their source can spot dangerous behaviors and detect individuals along with dangerous elements through on-site processing.
This eliminates sensitive data from cloud transfers thereby increasing protective measures and resolving privacy questions. Security personnel aided by AI-driven edge systems make faster and effective emergency responses when their systems analyze real-time drone, camera and sensor data in emergency situations.
Healthcare:
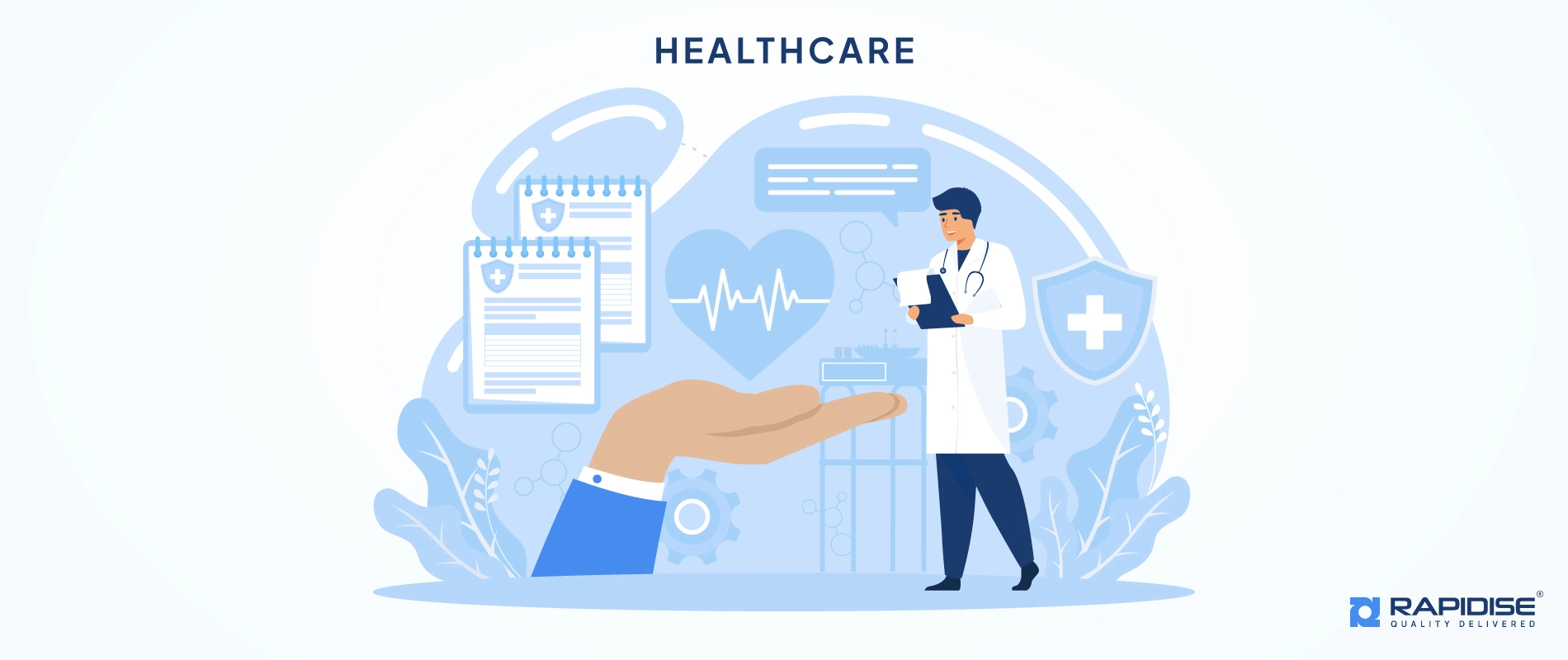
The combination of medical wearables with edge processors operating under AI algorithms maintains continuous health sign tracking of heart rate and oxygen levels and glucose levels together with blood pressure measurements. The devices evaluate healthcare data at their location before warning medical staff about abnormalities thus minimizing needless cloud data transfers yet still providing swift clinical responses. The system proves beneficial for monitoring patients who suffer from diabetes in addition to patients with cardiovascular disorders.
Edge AI diagnostic tools evaluate X-rays and MRIs and CT scans in real time through medical imaging to provide quick and precise diagnoses though they do not need cloud-based AI models. These conditions produce significant benefits for settings that face unstable internet functionality such as rural and underdeveloped regions.
Medical staff can obtain instant and accurate treatment information through edge computing even when a facility has restricted cloud connection capabilities. Multicomponent healthcare systems that use edge computing for processing medical data during real-time operations minimize dependency on cloud servers while improving operational productivity in medical facilities.
Manufacturing:
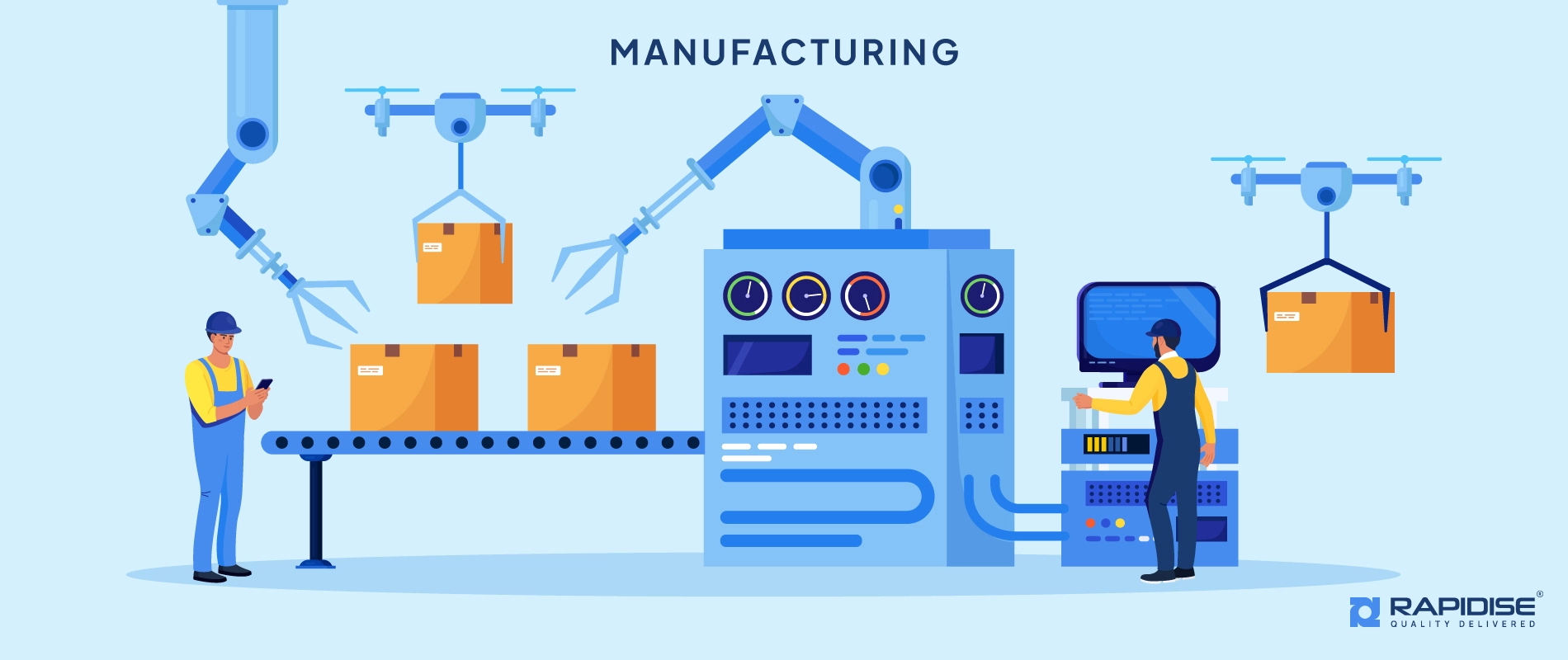
The most impactful edge computing application happens through predictive maintenance. These industrial machines benefit from edge sensors with AI models which analyze performance data to identify equipment failure symptoms before maintenance is scheduled before equipment breakdown occurs.
Equipment performance and reliability improve through this method because breakdowns are prevented while maintenance expenses decrease, and longevity of equipment increases. Edge AI operates as an essential factor to achieve quality control standards.
AI-powered vision systems with cameras function on assembly lines to check products while they detect both irregularities and defects and inconsistencies simultaneously. Manufacturers benefit from local processing with their systems since it lets them fix errors right away without waiting on cloud-based analysis thus delivering improved production quality.
Edge AI technology improves robotic systems along with automation protocols that operate in manufacturing facilities. Smart robots that use edge AI models can adjust to different settings and boost operation efficiency while teaming up with people in manufacturing production even when cloud connections are not available. People working in critical environments with strict timing requirements such as production lines and hazardous material handling benefit greatly from this particular feature.
Retail:
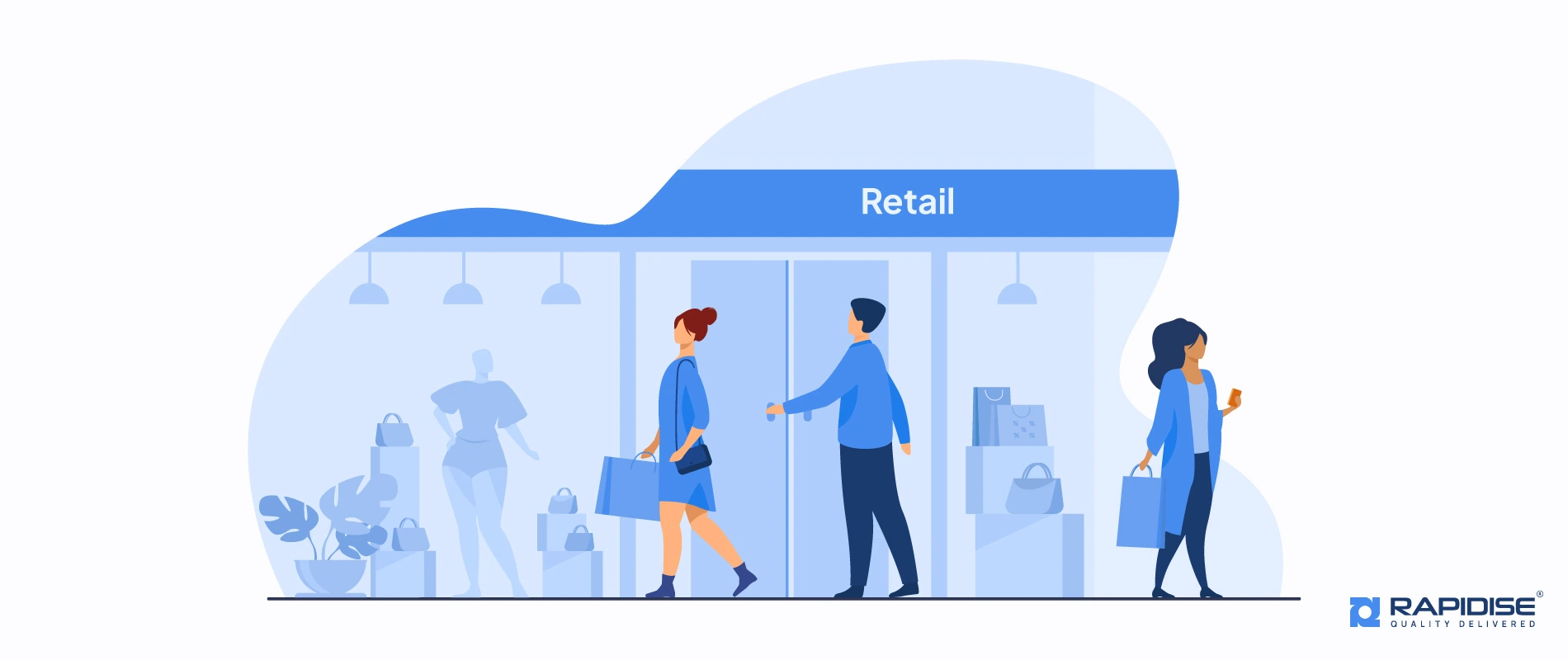
Modern smart retail technology consisting of AI-based video cameras together with IoT sensors performs real-time evaluation of shopping patterns and product selection behavior and purchase records of customers. Edge AI processes stored data in real-time which enables shops to provide personalized marketing offers together with product advice and variable pricing adjustments without moving customer information to remote servers.
Edge AI plays an essential role in inventory management by bringing numerous advantages to this field. An edge-based RFID scanning system and smart shelves combined measure inventory in real time thus stores can restock products right away without delays affecting supply chain excellence. The edge-based distribution of these systems removes time delays linked to cloud inventory tracking so stock information remains precise and current.
Please note that the application of customer analytics through edge AI helps retailers redesign layouts better while improving their relationships with consumers. AI foot traffic analysis provides retailers with insights into customer store movements which enables them to place high-demand products optimally for better customer experiences.
Conclusion
The future depicts a smarter and more scalable domain because AI model optimization and federated learning technologies and edge hardware specialize to overcome device management and security risks as well as hardware limitations and complexity during model deployment. Businesses should focus on selecting appropriate AI-powered edge technologies while establishing effective strategies because this will produce new opportunities for automation and digital transformation and operational efficiency.