Introduction
ADAS is one of the most transformational advanced technologies driving the automotive industry towards a revolution. ADAS image processing algorithms are used by these systems to enhance vehicle safety, efficiency and convenience. These algorithms are helping identify road signs, directing drivers to parking locations and more; they’re essential in helping make ADAS technologies a reality.
ADAS system processes data from cameras, RADAR and other sensors to ensure your safer and more efficient driving experience. In this blog, we look at how these algorithms are affecting and changing the automotive industry considering the challenges and solutions in their realization.
According to Statista Research Department, the global advanced driver assistance systems (ADAS) industry in 2024 raked in almost 58 billion U.S. dollars. The global ADAS market is about to grow to above 145 billion U.S. dollars by 2029. Those are some examples of the advanced driver assistance systems that include automated emergency braking, lane keep assist, automatic parking and adaptive cruise control.
ADAS system processes data from cameras, RADAR and other sensors to ensure your safer and more efficient driving experience. In this blog, we look at how these algorithms are affecting and changing the automotive industry considering the challenges and solutions in their realization.
According to Statista Research Department, the global advanced driver assistance systems (ADAS) industry in 2024 raked in almost 58 billion U.S. dollars. The global ADAS market is about to grow to above 145 billion U.S. dollars by 2029. Those are some examples of the advanced driver assistance systems that include automated emergency braking, lane keep assist, automatic parking and adaptive cruise control.
Road Sign Detection
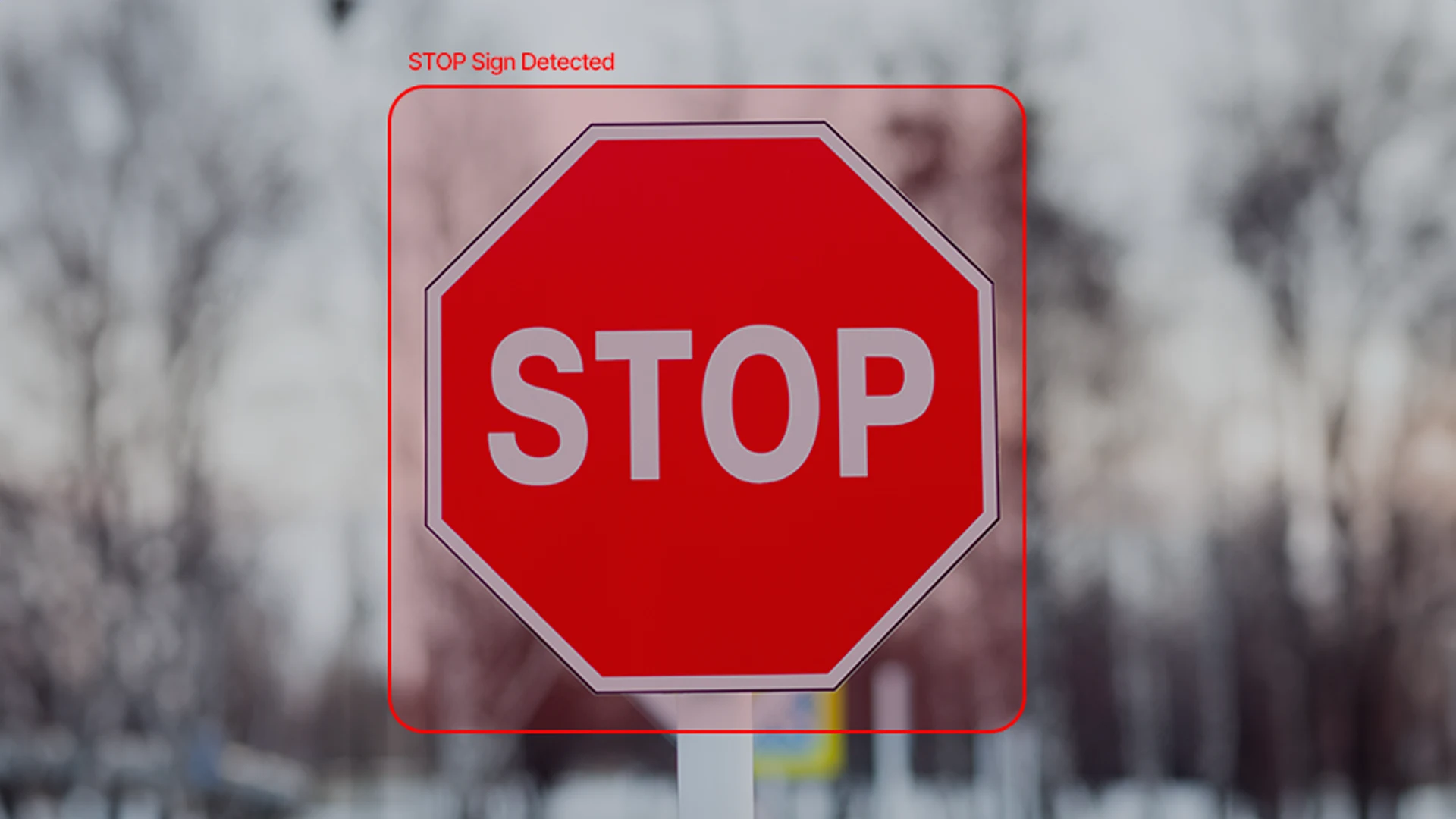
Detection of road signs is one of the primary features of ADAS technologies. Using such vision based ADAS systems, it recognizes traffic signs and provides critical information to drivers. For instance, real time speed limits, no entry signs or stop signs can control traffic in order to prevent penalties on behalf of the drivers. The advanced ADAS image processing algorithms help modern cars to accurately recognize signs, even at high speeds that make this feature indispensable.
Challenges and Solutions
Road sign detection is a challenging problem due to variability in sign design between regions and visibility in adverse weather conditions. Things become even more confusing if the signs on the road are blurred or partially obscured.
Combining robust ADAS image processing algorithms with machine learning models that will train systems over diverse datasets is the solution. It also leverages the ADAS systems using RADAR sensors and vision sensors to attain a higher accuracy in detection under difficult conditions. In addition, predictive analytics can help improve systems to understand which road signs they could infer even in areas where there were no visible road signs due to cracked or missed markers.
Combining robust ADAS image processing algorithms with machine learning models that will train systems over diverse datasets is the solution. It also leverages the ADAS systems using RADAR sensors and vision sensors to attain a higher accuracy in detection under difficult conditions. In addition, predictive analytics can help improve systems to understand which road signs they could infer even in areas where there were no visible road signs due to cracked or missed markers.
Pedestrian Detection
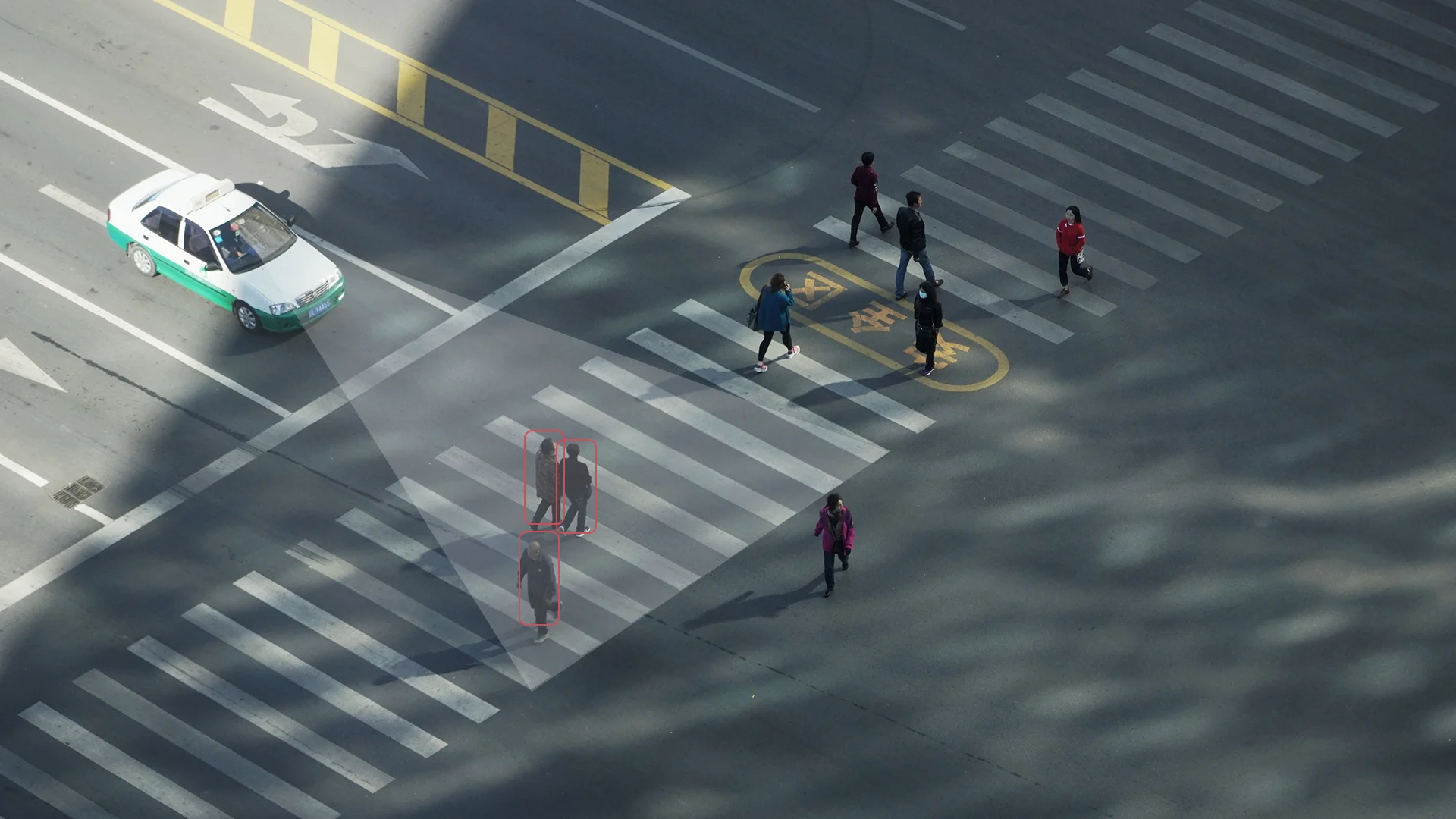
Pedestrian detection may prevent accidents by detecting pedestrians present or near the road. Interested in ADAS systems that provide timely warnings or automatic braking when necessary. Vision based ADAS systems process camera feeds to distinguish pedestrians from other objects. Such a feature is important for urban settings where pedestrians’ conduct is high.
Challenges and Solutions
The challenges involve detecting pedestrians in crowded urban scenes or under unsuitable conditions such as low light. As per solutions, RADAR based ADAS systems can be used to complement the cameras’ feeds to give 360 degree coverage as well as better object recognition.
Furthermore, integrating deep learning models improves the system in classifying between pedestrians and non-pedestrian objects. In fact, pedestrian detection can also be advanced to greater reliability in low light or night conditions through the use of advanced infrared sensors.
Furthermore, integrating deep learning models improves the system in classifying between pedestrians and non-pedestrian objects. In fact, pedestrian detection can also be advanced to greater reliability in low light or night conditions through the use of advanced infrared sensors.
Drive Innovation with Advanced ADAS Solutions
Apply the full power of ADAS image processing algorithms to disrupt the safety and efficiency of your vehicles once and for all. Join us and stay on top in the automotive world.
`Lane Keep Assist
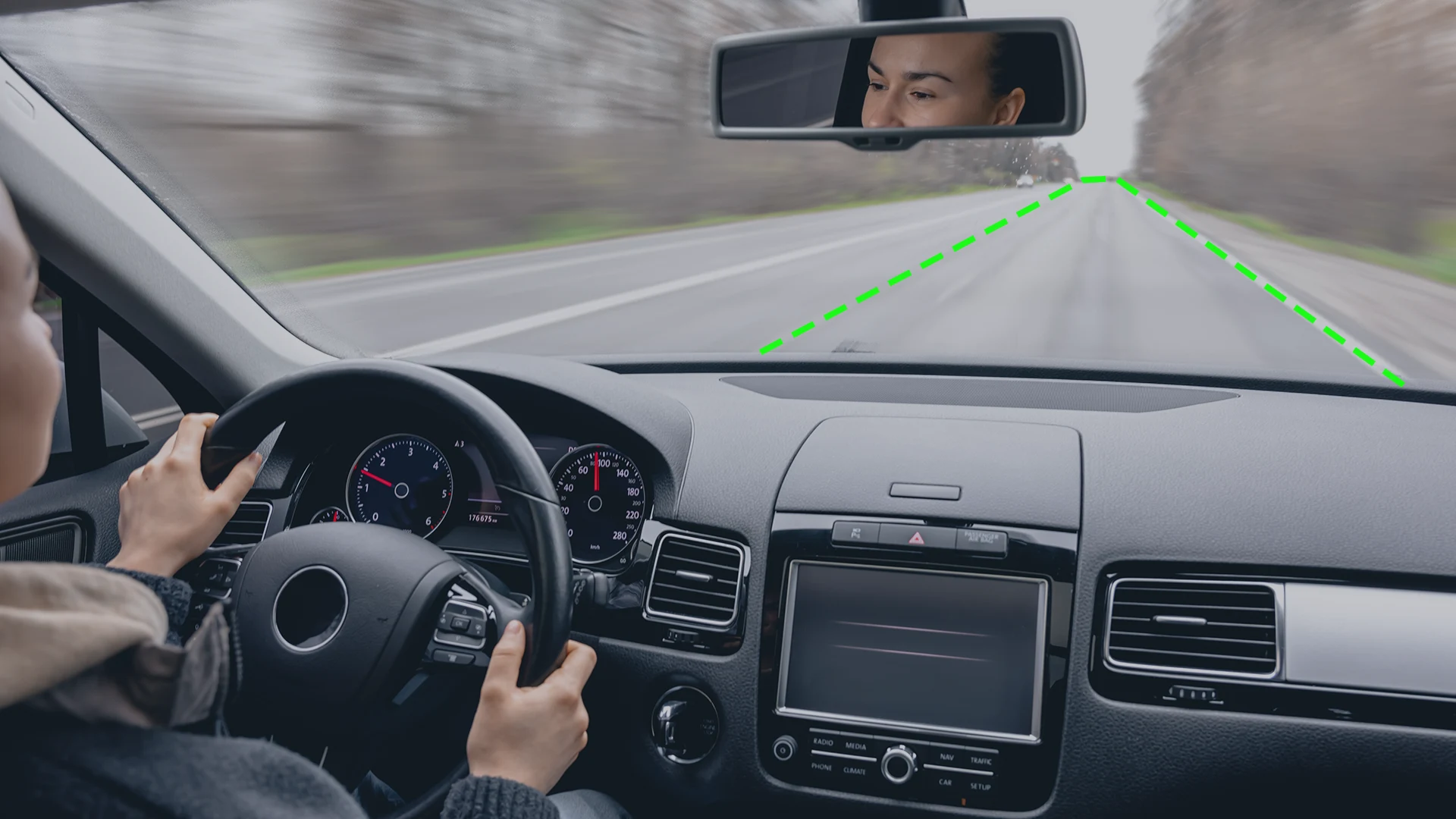
Lane Keep Assist (LKA) makes sure that a vehicle stays in lane by monitoring lane embossing. This function enabled by ADAS image processing algorithms informs the driver or steers the vehicle autonomously back into its lane in case of deviation. Long drives are especially helped here in reducing fatigue related lane departures.
Challenges and Solutions
This is very difficult for badly plotted roads and sometimes for temporary lane changes due to construction. This can be mitigated using high-definition cameras combined with advanced algorithms which predict lane boundaries.
Additionally, RADAR based ADAS systems enhance detection in adverse weather conditions. Systems also can incorporate GPS data and a digital road map for a more reliable addition of lane prediction accuracy.
Additionally, RADAR based ADAS systems enhance detection in adverse weather conditions. Systems also can incorporate GPS data and a digital road map for a more reliable addition of lane prediction accuracy.
Blind Spot Monitoring
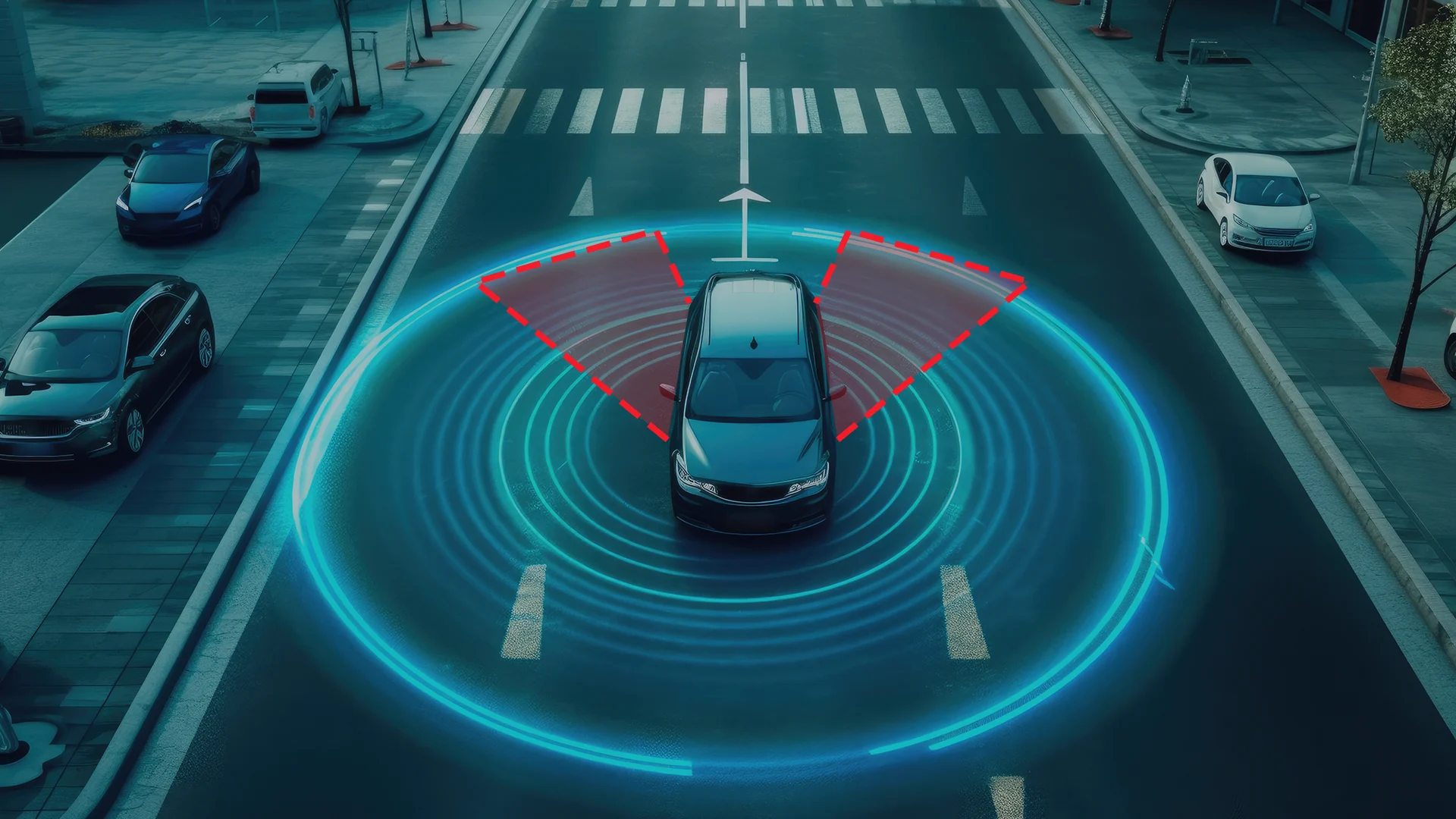
Blind Spot monitoring (BSM) systems insure from facing accidents due to a car in the blind spot of a vehicle. Combining vision based ADAS systems and RADAR based ADAS systems, the advanced driver assistance and early warning technology detects the vehicles in the adjacent lanes in real time and alerts the drivers. In modern vehicles this feature has become a standard safety element.
Challenges and Solutions
Fast approaching vehicles or small objects/bicycles cannot be detected in real time. For this reason, solutions are provided in the integration of Artificial Intelligent powered ADAS image processing algorithms capable of differentiating between objects by speed and size.
Adding enhanced sensor fusion such as combining data from cameras, RADAR and ultrasonic sensors will decrease the detection accuracy even further. Furthermore, the 3D imaging system deployment can provide a more complete view of surroundings for a vehicle.
Adding enhanced sensor fusion such as combining data from cameras, RADAR and ultrasonic sensors will decrease the detection accuracy even further. Furthermore, the 3D imaging system deployment can provide a more complete view of surroundings for a vehicle.
Driver Authentication and Status Monitoring (DASM)
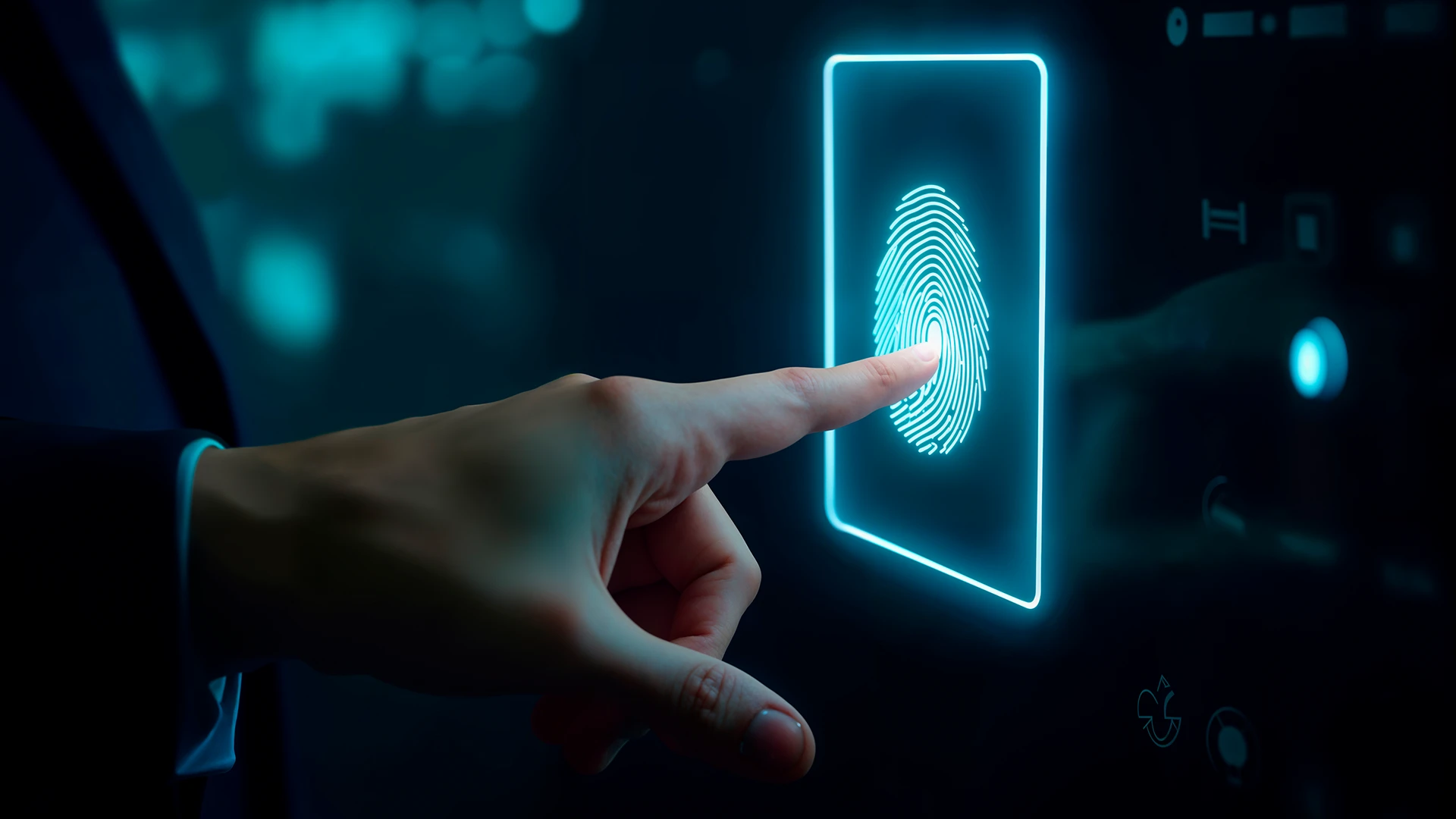
By screening for fatigue, distractions and intoxication among drivers, DASM assures driver safety. The ADAS technologies based on vision assess facial cues and eye movement to determine the condition of a driver. Human error causing accidents has been reduced with this feature.
Challenges and Solutions
This places the task of detecting subtle changes in driver behavior and achieving accuracy across a variety of demographics and lighting conditions, as significant hurdles. These limitations are addressed by high resolution cameras combined with a suite of advanced ADAS image processing algorithms.
AI led adaptive learning models are best suited to recognize data from individuals with individual variations and external factors. Finally, DASM can be integrated biometric authentication systems which verify driver identity and monitor health conditions.
AI led adaptive learning models are best suited to recognize data from individuals with individual variations and external factors. Finally, DASM can be integrated biometric authentication systems which verify driver identity and monitor health conditions.
Forward and Backward Collision Warnings (FBCW)
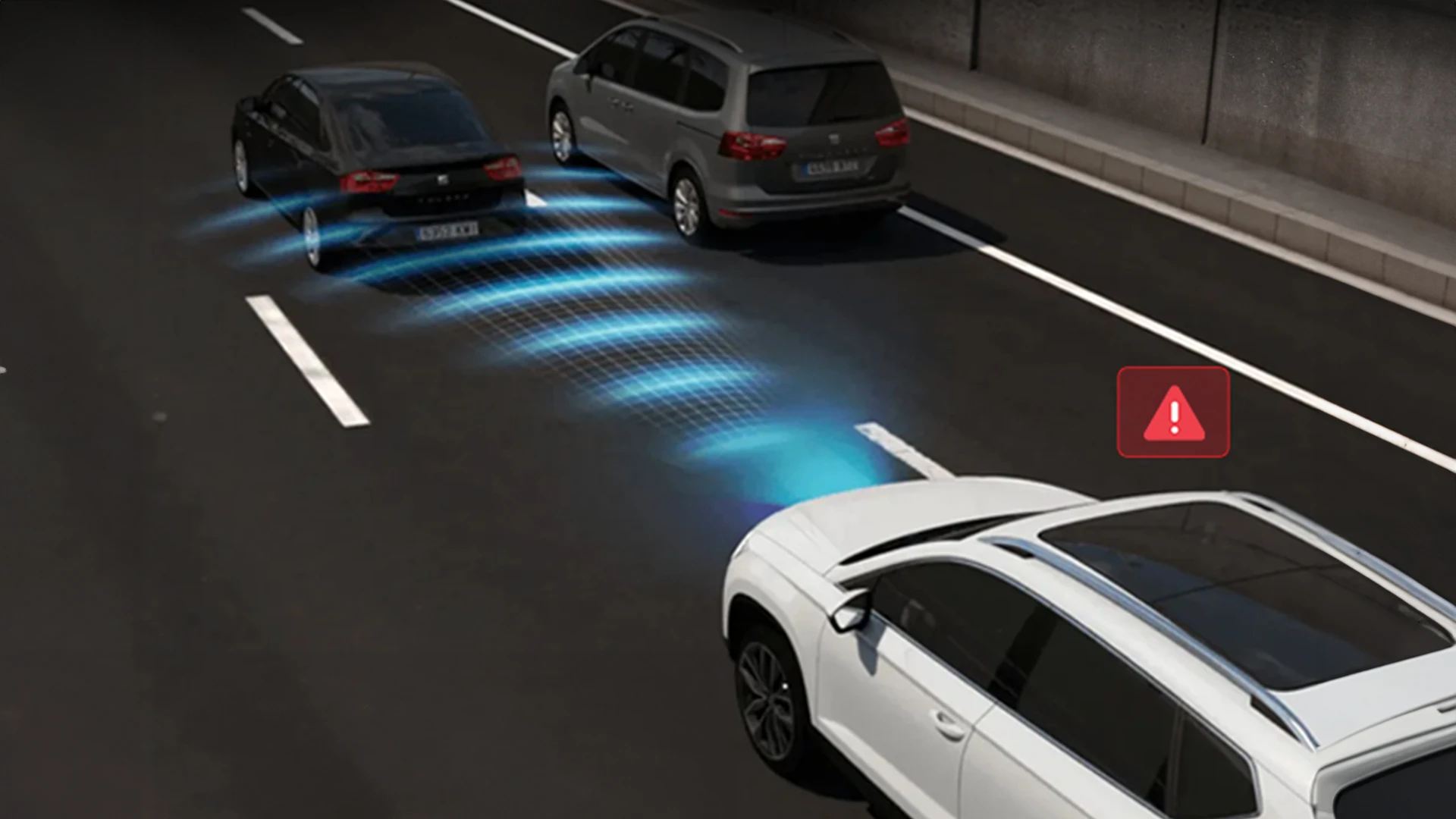
FBCW systems identify obstacles in the vehicle path in order to prevent accidents. The vision based ADAS systems, and the RADAR based ADAS systems present real time alerts or automatically brakes when needed. It dramatically enhances safety in urban and highway conditions.
Challenges and Solutions
Key challenges include detecting small objects at high speeds or discriminating stationery from mobile obstacles. The solutions are to use RADAR-based ADAS systems for long range detection and AI enhanced algorithms for better object classification.
Multiple sensor inputs are combined to provide redundancy as well as accuracy. Further improvement of the system’s ability to deal with dynamic environments is possible with advanced motion prediction algorithms.
Multiple sensor inputs are combined to provide redundancy as well as accuracy. Further improvement of the system’s ability to deal with dynamic environments is possible with advanced motion prediction algorithms.
Parking Assistance
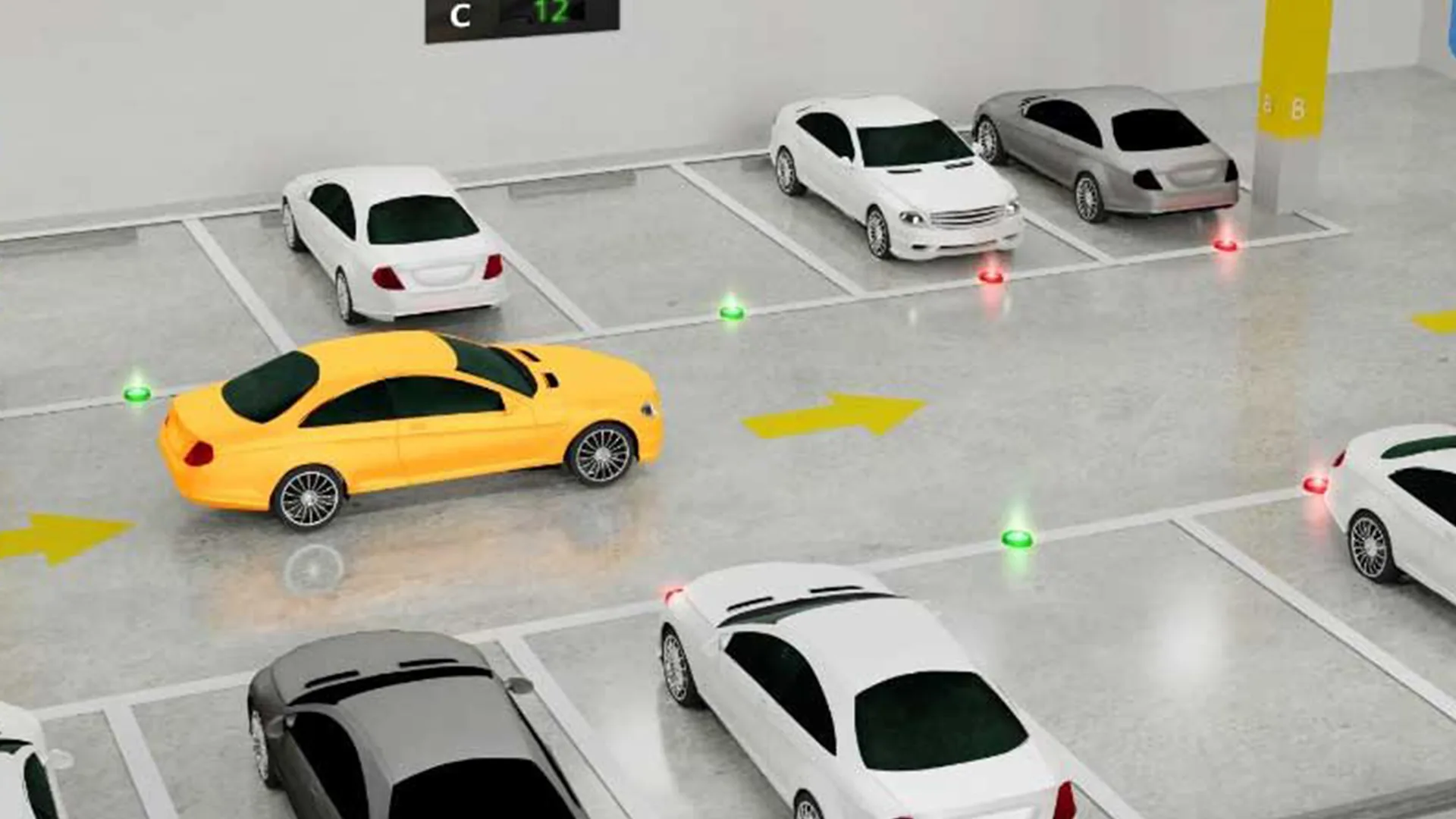
The parking assistance systems make running the usually difficult job of parking much easier. The vision based ADAS system uses the data coming from cameras and sensors to provide parallel or perpendicular parking guidance. In crowded urban areas, where there are very little spaces for parking, these systems prove particularly useful.
Challenges and Solutions
Because cars have limited visibility in tight parking spaces and are not always capable of seeing what our previously stated object examples would be (such as a curb) … The combined use of ultrasonic sensors with advanced ADAS image processing algorithms allow them to provide a more complete view of the environment.
Moreover, machine learning will enable real time predictive modeling of precise parking maneuvers. Better of parking assistance system is provided by incorporating 360 degree camera system which further improves the reliability of parking assistance system.
Moreover, machine learning will enable real time predictive modeling of precise parking maneuvers. Better of parking assistance system is provided by incorporating 360 degree camera system which further improves the reliability of parking assistance system.
Transform Your Automotive Systems with ADAS Technologies
Find cutting edge ADAS development services that improve driver safety and vehicle performance. Learn how our solutions can help you succeed.
Vehicle Functions Control
There are different vehicle functions that ADAS systems will have the ability to control, such as adaptive cruise control and automatic emergency braking. Real time data is processed by ADAS image processing algorithms to optimize the functioning of these functions to achieve a smoother and a safer driving experience. For semi autonomous and fully autonomous operations, these controls are critical.
Challenges and Solutions
However, there are many challenges including system latency and compatibility of the system with different vehicle models. Improving software scalability and implementing RADAR based ADAS systems leading to quicker data acquisition are involved with solutions.
Further, real time computing frameworks cut latency to the point where instant responses are possible. Advanced over the air (OTA) update mechanisms can ensure that compatibility and up to date systems can be achieved.
Further, real time computing frameworks cut latency to the point where instant responses are possible. Advanced over the air (OTA) update mechanisms can ensure that compatibility and up to date systems can be achieved.
Vehicle Classification
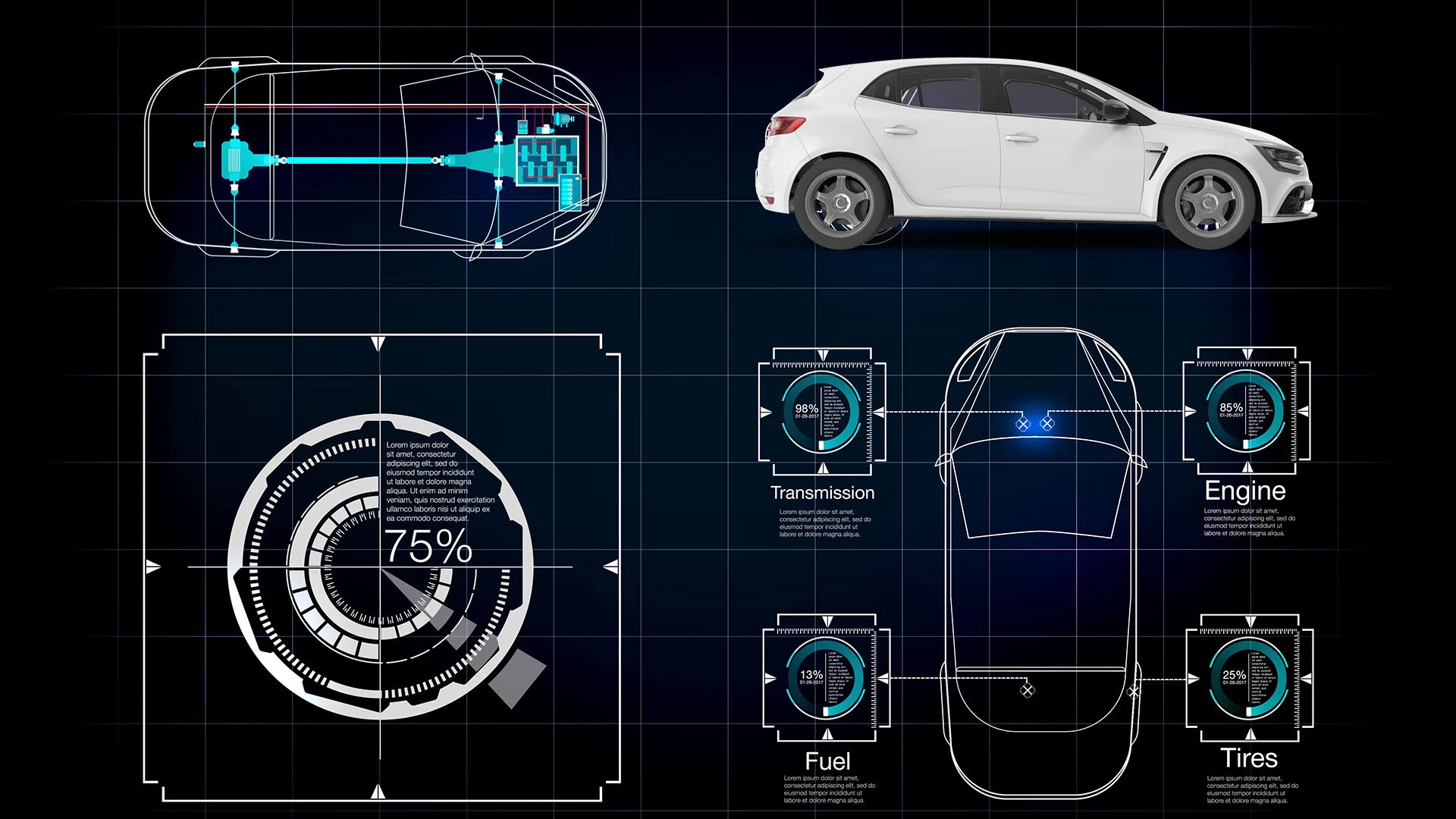
Applications — such as traffic monitoring and toll collection — depend on vehicle classification. ADAS image processing algorithms inspect the vehicle dimensions, shapes and speeds to clearly classify. This capability also can be used for traffic flow optimization and road infrastructure planning.
Challenges and Solutions
It is challenged by misclassification arising from overlapping vehicles or poor lighting. The solution to this is to merge the benefits of both AI driven image processing and 3D modeling, making the results more accurate.
Using vision based ADAS systems and RADAR increases reliability of the system under different conditions. Further edge computing improves both the system efficiency and responsiveness of implementing edge computing for real time processing.
Using vision based ADAS systems and RADAR increases reliability of the system under different conditions. Further edge computing improves both the system efficiency and responsiveness of implementing edge computing for real time processing.
Conclusion
The automotive industry is undergoing a paradigm shift through ADAS image processing algorithms. These algorithms are used to ensure safer, up to more efficient driving experiences from vehicle classification to road sign detection. The automotive industry is aiding challenges and leading the future autonomous and assisted driving by enabling RADAR based ADAS systems and using vision based ADAS technology.
Going forward, as ADAS development advances, integrating AI and machine learning will only continue to increase the accuracy, reliability and adaptability of these systems which results in safer roads for everyone. Additionally, due to the convergence of these technologies with IoT and 5G connectivity, ADAS systems have greater potential which will lead to innovation and mobility is the byproduct.
Going forward, as ADAS development advances, integrating AI and machine learning will only continue to increase the accuracy, reliability and adaptability of these systems which results in safer roads for everyone. Additionally, due to the convergence of these technologies with IoT and 5G connectivity, ADAS systems have greater potential which will lead to innovation and mobility is the byproduct.